Epidemic situation prediction method, device and equipment based on ensemble learning model, and medium
A technology of integrated learning and prediction methods, applied in the field of devices, epidemic prediction methods based on integrated learning models, electronic equipment and storage media, can solve the problems of matching epidemic data, unsatisfactory prediction effects, and affecting the accuracy of epidemic prediction results, etc. Achieving the effect of avoiding influence and strong adaptability
- Summary
- Abstract
- Description
- Claims
- Application Information
AI Technical Summary
Problems solved by technology
Method used
Image
Examples
Embodiment 1
[0062] Embodiment 1 provides an epidemic prediction method based on an integrated learning model, which aims to train an integrated learning model including a logistic growth model and a neural network model through epidemic data processed in a time dimension, and further automatically select a prediction model based on the loss value , And then accurately predict the epidemic trend. This method effectively avoids the impact on the epidemic prediction results caused by the time difference in data acquisition, and the integrated learning model adopted is highly adaptable, and can be quickly fine-tuned according to the real-time epidemic data, so that the prediction model selected adaptively according to the loss value can be Accurately make dynamic predictions of future epidemic trends, and the prediction results are in line with actual epidemic development trends.
[0063] Please refer to figure 1 As shown, an epidemic prediction method based on an integrated learning model incl...
Embodiment 2
[0089] The second embodiment is an improvement based on the first embodiment, please refer to figure 2 As shown, according to the epidemic data sequence X in the training set M1 M1 , Train the logistic growth model and V neural network models separately, obtain the trained logistic growth model and the trained V neural network models, and then make the integrated learning model integrate the logistic growth model's characteristics in line with the epidemic development law and neural The network model has the advantages of data abstraction capabilities, so as to more accurately predict the development trend of the epidemic.
[0090] S1301 According to the epidemic data sequence X in the training set M1 M1 , Training logistic growth model.
[0091] There is an incubation period in the epidemic, and there is a certain time difference from infection to suspected, confirmed, cured or accurate judgments of death. In order to avoid the time difference affecting the accuracy of the epidemi...
Embodiment 3
[0108] The third embodiment is a specific application embodiment corresponding to the above-mentioned embodiment 1. This embodiment uses the cumulative number of confirmed diagnoses of 2019nCov nationwide from February 1, 2020 to February 22, 2020, and obtains the prediction model 0222 and calculates it on February 25, 2020. The development trend of the epidemic from January to March 2, 2020 is predicted. The cumulative number of confirmed diagnoses in 2019nCov from February 1 to February 22, 2020 is shown in the table below.
[0109] Table 1. The cumulative number of confirmed cases of 2019nCov nationwide from February 1, 2020 to February 22, 2020
[0110] date The cumulative number of confirmed cases nationwide February 111891 February 214490 February 317341 February 420530 February 524434 February 628138 February 731264 February 834673 February 937289 February 1040262 February 1142747 February 1244765 February 1359907 February 1463950 February 1566581 Februa...
PUM
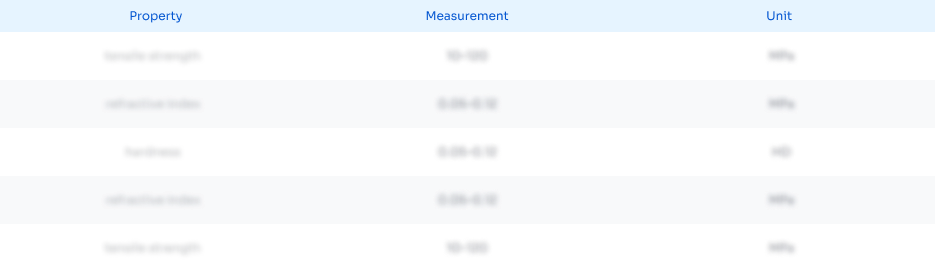
Abstract
Description
Claims
Application Information
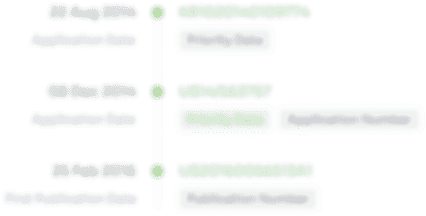
- R&D Engineer
- R&D Manager
- IP Professional
- Industry Leading Data Capabilities
- Powerful AI technology
- Patent DNA Extraction
Browse by: Latest US Patents, China's latest patents, Technical Efficacy Thesaurus, Application Domain, Technology Topic, Popular Technical Reports.
© 2024 PatSnap. All rights reserved.Legal|Privacy policy|Modern Slavery Act Transparency Statement|Sitemap|About US| Contact US: help@patsnap.com