PCR prediction method based on multi-type medical data
A technology for medical data and prediction methods, applied in medical images, healthcare informatics, informatics, etc., can solve the problems of reducing model prediction speed, reducing prediction performance, affecting prediction effect, etc., to improve accuracy and efficiency, improve Efficiency and Accuracy Improvement
- Summary
- Abstract
- Description
- Claims
- Application Information
AI Technical Summary
Problems solved by technology
Method used
Image
Examples
Embodiment Construction
[0018] The following will clearly and completely describe the technical solutions in the embodiments of the present invention with reference to the accompanying drawings in the embodiments of the present invention. Obviously, the described embodiments are only some, not all, embodiments of the present invention. Based on the embodiments of the present invention, all other embodiments obtained by persons of ordinary skill in the art without creative efforts fall within the protection scope of the present invention.
[0019] figure 1 It is an overall flowchart of the pCR prediction method based on multi-type medical data of the embodiment of the present invention, such as figure 1 As shown, the method includes:
[0020] S1, obtain clinical data, CT diagnosis report and colonoscopy image from the medical department, preprocess these three kinds of medical data, output the characteristics of normalized clinical data, CT diagnosis report represented by fixed-length vector, and fix...
PUM
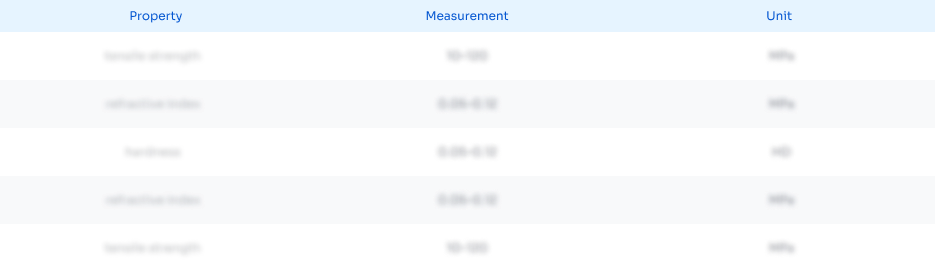
Abstract
Description
Claims
Application Information
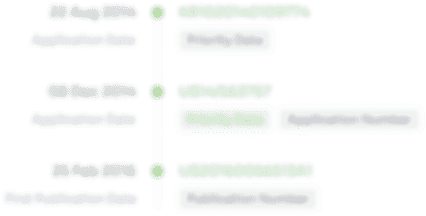
- R&D Engineer
- R&D Manager
- IP Professional
- Industry Leading Data Capabilities
- Powerful AI technology
- Patent DNA Extraction
Browse by: Latest US Patents, China's latest patents, Technical Efficacy Thesaurus, Application Domain, Technology Topic, Popular Technical Reports.
© 2024 PatSnap. All rights reserved.Legal|Privacy policy|Modern Slavery Act Transparency Statement|Sitemap|About US| Contact US: help@patsnap.com