Logging curve prediction method based on modal decomposition reconstruction and depth LSTM-RNN model
A well logging curve and modal decomposition technology, applied in deep learning methods and petroleum geophysical prospecting fields, can solve problems such as non-stationarity and strong nonlinearity of underutilized well logging curves
- Summary
- Abstract
- Description
- Claims
- Application Information
AI Technical Summary
Problems solved by technology
Method used
Image
Examples
Embodiment Construction
[0081] In order to make the purpose, technical solutions and advantages of the embodiments of the present invention clearer, the technical solutions in the embodiments of the present invention will be clearly and completely described below. Obviously, the described embodiments are some embodiments of the present invention, not all the embodiment. It should be noted that representation and description of components and processes that are not related to the present invention and that are known to those of ordinary skill in the art are omitted from the description for the purpose of clarity. Based on the embodiments of the present invention, all other embodiments obtained by persons of ordinary skill in the art without making creative efforts belong to the protection scope of the present invention.
[0082] A logging curve prediction method based on modal decomposition reconstruction and deep LSTM-RNN model, see figure 1 and image 3 , including CEEMD decomposition, run length de...
PUM
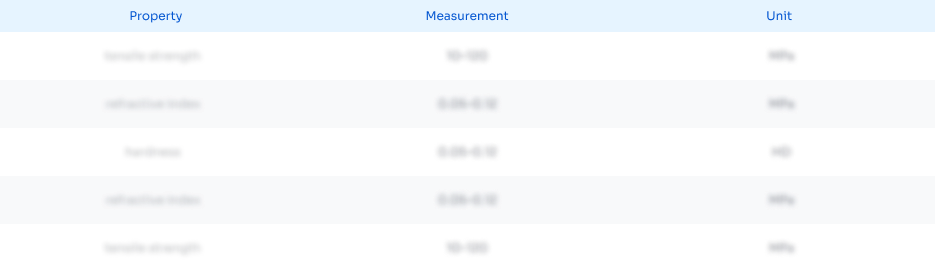
Abstract
Description
Claims
Application Information
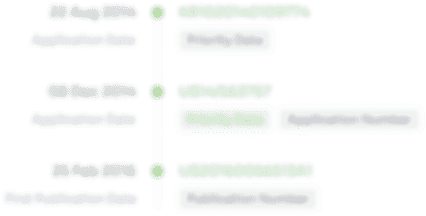
- R&D Engineer
- R&D Manager
- IP Professional
- Industry Leading Data Capabilities
- Powerful AI technology
- Patent DNA Extraction
Browse by: Latest US Patents, China's latest patents, Technical Efficacy Thesaurus, Application Domain, Technology Topic, Popular Technical Reports.
© 2024 PatSnap. All rights reserved.Legal|Privacy policy|Modern Slavery Act Transparency Statement|Sitemap|About US| Contact US: help@patsnap.com