Method for improving data clustering quality by improving k-means based on deviation maximization method
A technology of maximizing deviation and data clustering, applied in the field of data processing, can solve problems such as the selection of K value that cannot be solved, and achieve fast speed and good effect
- Summary
- Abstract
- Description
- Claims
- Application Information
AI Technical Summary
Problems solved by technology
Method used
Image
Examples
Embodiment 1
[0039] A method for improving the quality of data clustering based on the method of maximizing deviation to improve k-means, including:
[0040] 1) Calculate the weight of the maximum deviation of the read data:
[0041] Objective weight ω j The formula for determining the method is as follows:
[0042]
[0043] In formula (1), i and j represent rows and columns. The objective weight can make full use of the information of the decision object, and widen the gap between data in order to make better decisions;
[0044] 2) Use the maximum dispersion method to calculate the weight w of each attribute of the sample k , And then construct a weighted matrix, and construct a matrix according to the sample data arrangement;
[0045] 3) Weight the attributes of the data set:
[0046] Set data attribute X = {x i1 ,x i2 ,...,x im }, where m represents the number of attributes, divide these data into k classes, and the attribute weight value is w 1 ,w 2 ,...,w m , And w j > = 0, j = 1, 2,..., m, the...
Embodiment 2
[0051] As described in Example 1, a method for improving the quality of data clustering based on the method of maximizing dispersion to improve k-means, the difference is that the k-means++ algorithm specifically includes:
[0052] Assuming that the data sample set is divided into k categories, the steps of the k-means++ algorithm are as follows:
[0053] 1) Randomly select the first center point and preset a value of k, where k is the number of categories;
[0054] 3) Calculate the shortest distance between each element and each center point, denoted by D(X); among them, the larger the point of D(X), the greater the probability that the center point is, until k center points are found;
[0055] 3) After the cth iteration, for any sample data, find the distance to the k centers, and then classify the sample into the cluster where the center with the shortest distance is located;
[0056] 4) Use the mean value calculation method to update the center value of these clusters;
[0057] 5) Fo...
experiment example
[0059] Attached figure 1 , To verify the effect of the present invention.
[0060] This experimental example is to use the method of the present invention to improve the k-means based on the dispersion maximization method to improve the quality of data clustering, respectively, to perform data on the two representative data sets Iris and Wine in the UCI machine learning database. Cluster processing, and then visually check the data clustering quality.
[0061] Among them, the UCI database is a database specially used for testing machine learning and data mining algorithms. The data in the database has a certain classification, so the quality of the clustering can be seen intuitively. The data sets Iris and Wine, as experimental data, are shown in Table 3.1 Experimental Data Sets. The Iris data set contains 150 labeled 4-dimensional sample points, and the number of cluster categories is k=3; the Wine data set has 178 items, and 13 parameters are used to distinguish 3 wine varieti...
PUM
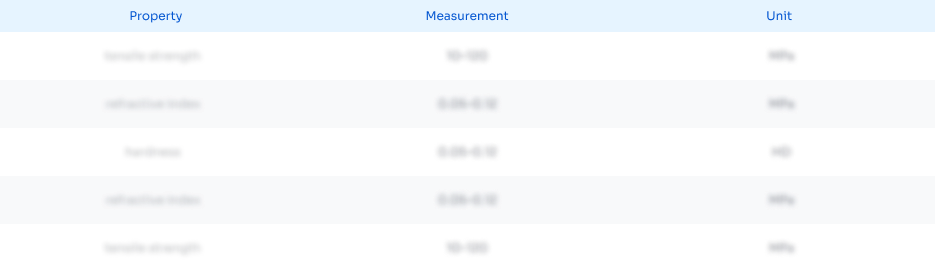
Abstract
Description
Claims
Application Information
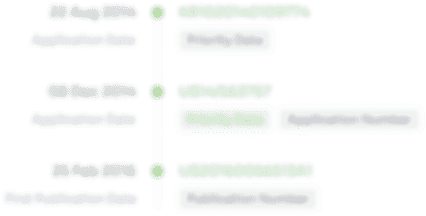
- R&D Engineer
- R&D Manager
- IP Professional
- Industry Leading Data Capabilities
- Powerful AI technology
- Patent DNA Extraction
Browse by: Latest US Patents, China's latest patents, Technical Efficacy Thesaurus, Application Domain, Technology Topic, Popular Technical Reports.
© 2024 PatSnap. All rights reserved.Legal|Privacy policy|Modern Slavery Act Transparency Statement|Sitemap|About US| Contact US: help@patsnap.com