An intelligent ensemble assessment method and system for basin water and sediment research models
A technology for studying models and watersheds, applied in the field of ensemble assessment, which can solve problems such as inability to guarantee, model structure errors, and inaccurate human activity contribution rates.
- Summary
- Abstract
- Description
- Claims
- Application Information
AI Technical Summary
Problems solved by technology
Method used
Image
Examples
Embodiment 1-4 and comparative example 1-2
[0096] Examples 1-4 and Comparative Examples 1-2: An ensemble evaluation method for research models of runoff change in the Huangfuchuan River Basin
[0097] The first step is to establish the research object: a study on the runoff change in the Huangfuchuan River Basin from 1982 to 2015, with a total of 408 samples. On the annual and monthly scales, this paper selects three methods: Multiple Linear Regression (MLR), kNN Regression (kNNR) and Support Vector Regression (SVR). The output variable is selected as runoff, and the input variables are precipitation, evapotranspiration, and human activities. There are three types of variables. The time scale is selected as annual scale and monthly scale, and the established research objects are shown in Table 1.
[0098] Table 1 Selection of variables related to water-sediment change in the Huangfuchuan River Basin
[0099]
[0100] The second step is to screen the optimal model: use three different machine learning methods to ob...
Embodiment 5-8 and comparative example 3-4
[0125] Examples 5-8 and Comparative Examples 3-4: An ensemble evaluation method for research models on sediment transport changes in the Huangfuchuan River Basin
[0126] The first step is to establish the research object: to study the change of sediment discharge in the Huangfuchuan River Basin, three models of multiple linear regression, kNN regression and support vector regression are selected on the annual and monthly scales.
[0127] The second step is to screen the optimal model: At the same time, different types of independent variable combinations are selected, and a total of 12 groups of sand transport models are trained, covering 12 situations, as shown in Table 6.
[0128] Table 6 Multi-angle research on sediment transport changes in the Huangfuchuan River Basin
[0129]
[0130]
[0131] Remarks: The monthly sediment discharge data from 1991 to 1994 are missing.
[0132] Models were generated in the same manner as in Examples 1-4, and an optimal model for ea...
PUM
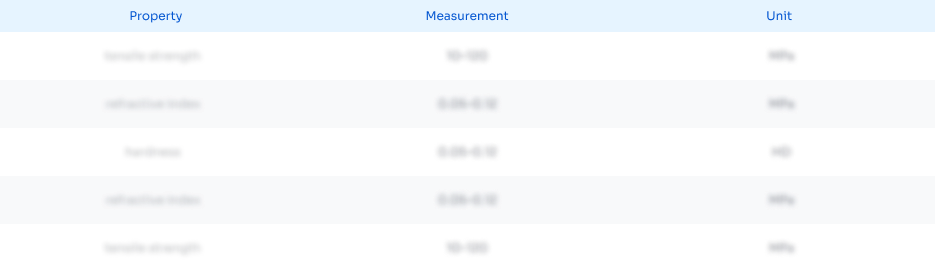
Abstract
Description
Claims
Application Information
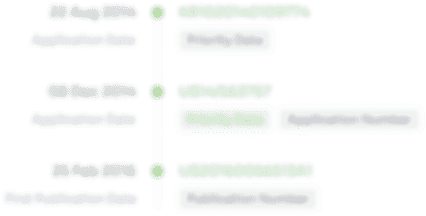
- R&D Engineer
- R&D Manager
- IP Professional
- Industry Leading Data Capabilities
- Powerful AI technology
- Patent DNA Extraction
Browse by: Latest US Patents, China's latest patents, Technical Efficacy Thesaurus, Application Domain, Technology Topic.
© 2024 PatSnap. All rights reserved.Legal|Privacy policy|Modern Slavery Act Transparency Statement|Sitemap