High-resolution remote sensing image classification method based on novel feature pyramid depth network
A feature pyramid and remote sensing image technology, applied in the field of high-scoring remote sensing image classification based on deep learning, can solve the problems of damaged high-frequency components of images, blurred edges of instance objects, and large amount of calculation.
- Summary
- Abstract
- Description
- Claims
- Application Information
AI Technical Summary
Problems solved by technology
Method used
Image
Examples
Embodiment Construction
[0091] The technical solutions of the present invention will be further described below in conjunction with the accompanying drawings and embodiments.
[0092] Such as figure 1 As shown, a further detailed description is as follows:
[0093] 1. Construct a multi-category remote sensing image dataset, and make corresponding sample labels, and divide each type of remote sensing image into a training set Train and a test set Test in proportion;
[0094] (1.1) Divide multi-category remote sensing image dataset Image=[Image 1 ,...,Image i ,...,Image N ], and make the corresponding sample label Label=[Label 1 ,...,Label i ,...,Label N ], where N means that there are N types of remote sensing images in total, and Image i Indicates the i-th type of remote sensing image set, Label i Represents the label set of the i-th type of remote sensing image, the value of the label set is i-1, and the value of i is i=1,2,...,N;
[0095] (1.2) Divide each type of remote sensing image data...
PUM
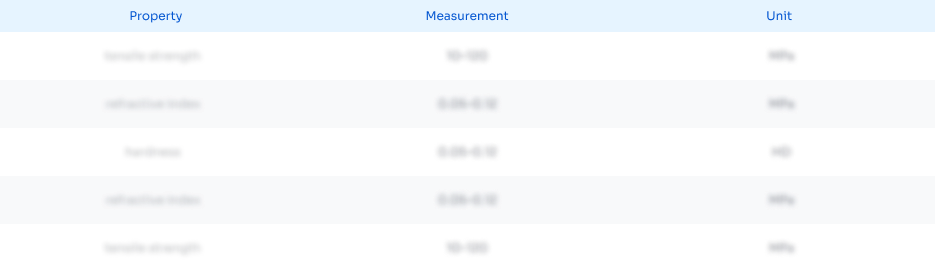
Abstract
Description
Claims
Application Information
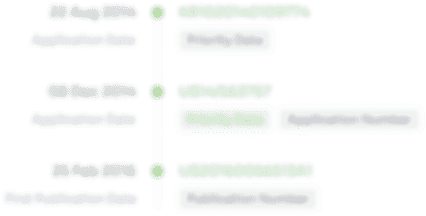
- Generate Ideas
- Intellectual Property
- Life Sciences
- Materials
- Tech Scout
- Unparalleled Data Quality
- Higher Quality Content
- 60% Fewer Hallucinations
Browse by: Latest US Patents, China's latest patents, Technical Efficacy Thesaurus, Application Domain, Technology Topic, Popular Technical Reports.
© 2025 PatSnap. All rights reserved.Legal|Privacy policy|Modern Slavery Act Transparency Statement|Sitemap|About US| Contact US: help@patsnap.com