Machine learning model robustness evaluation method based on noise data
A machine learning and robust technology, applied in the field of model robustness evaluation, can solve problems such as high cost and impact evaluation algorithm, and achieve the effect of comprehensive and perfect cognition
- Summary
- Abstract
- Description
- Claims
- Application Information
AI Technical Summary
Problems solved by technology
Method used
Image
Examples
Embodiment Construction
[0035] Embodiments of the present invention are described below through specific examples, and those skilled in the art can easily understand other advantages and effects of the present invention from the content disclosed in this specification.
[0036] Such as figure 1 As shown, the method for evaluating model robustness based on noise data in this embodiment mainly includes:
[0037] 1. Model the original data set to get the accuracy of the model. Among them, the original data is a data set with 100% correct labels, and the method of dividing the training set and the test set is 10 times of 10-fold cross-validation. Substituting the original training set into N different supervised learning algorithms, we train N different predictive models. Based on the original test set, we evaluate the accuracy of these N models and obtain the corresponding N accuracy rates.
[0038] 2. Model the noisy data to get the accuracy of the new model. Among them, on the basis of the origina...
PUM
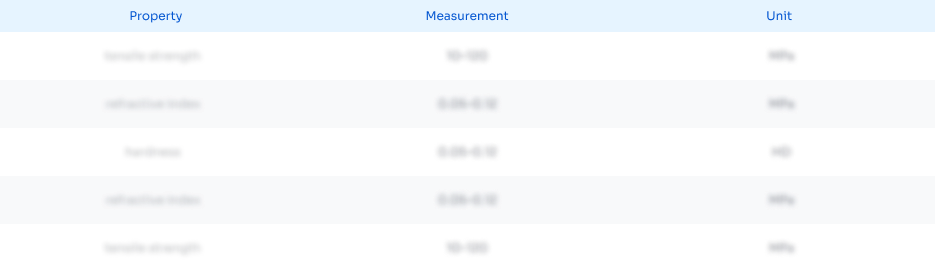
Abstract
Description
Claims
Application Information
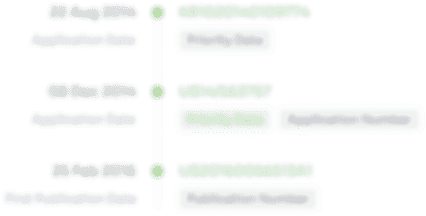
- R&D
- Intellectual Property
- Life Sciences
- Materials
- Tech Scout
- Unparalleled Data Quality
- Higher Quality Content
- 60% Fewer Hallucinations
Browse by: Latest US Patents, China's latest patents, Technical Efficacy Thesaurus, Application Domain, Technology Topic, Popular Technical Reports.
© 2025 PatSnap. All rights reserved.Legal|Privacy policy|Modern Slavery Act Transparency Statement|Sitemap|About US| Contact US: help@patsnap.com