A Personalized Movie Recommendation Method Based on Neural Network and Collaborative Filtering
A collaborative filtering algorithm and neural network technology, applied in the field of personalized recommendation, can solve problems such as the decline in the accuracy of feature extraction, the one-way and limitation of standard language models, etc.
- Summary
- Abstract
- Description
- Claims
- Application Information
AI Technical Summary
Problems solved by technology
Method used
Image
Examples
Embodiment Construction
[0040] The invention will be further described below in conjunction with the accompanying drawings and examples.
[0041] figure 1 A schematic diagram of the Bert neural network.
[0042] figure 2 A flowchart of the NLP process.
[0043] image 3 In order to compare the experimental errors of Bert-SVD and Funk-SVD, the present invention uses a group of data of 100,000 scores generated by 943 users and 1682 items provided by Movielens to test, and sets the implicit feedback dimension K to 100, and learns The rate is set to 0.002, the regularization parameter is set to 0.01, and the number of iterations is 800. The minimum error of the original Funk-SVD is 0.129, and the minimum error of the present invention is 0.126.
PUM
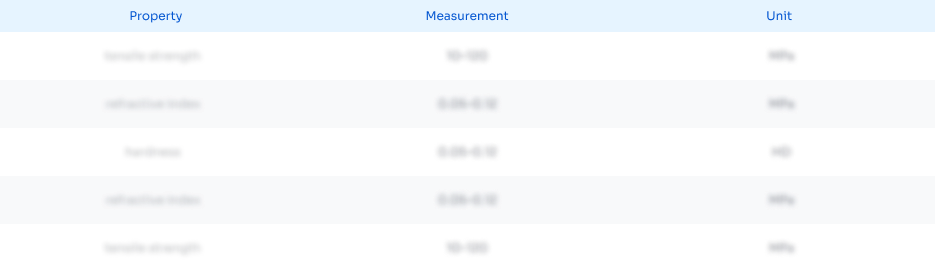
Abstract
Description
Claims
Application Information
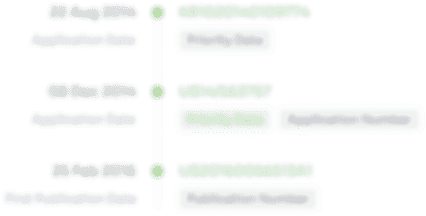
- R&D Engineer
- R&D Manager
- IP Professional
- Industry Leading Data Capabilities
- Powerful AI technology
- Patent DNA Extraction
Browse by: Latest US Patents, China's latest patents, Technical Efficacy Thesaurus, Application Domain, Technology Topic, Popular Technical Reports.
© 2024 PatSnap. All rights reserved.Legal|Privacy policy|Modern Slavery Act Transparency Statement|Sitemap|About US| Contact US: help@patsnap.com