Short-term impact load prediction method based on two-layer decomposition technology
A technology of shock load and prediction method, which is applied in the field of short-term shock load prediction based on two-layer decomposition technology, and can solve the problem of inaccurate prediction results due to nonlinear characteristics
- Summary
- Abstract
- Description
- Claims
- Application Information
AI Technical Summary
Problems solved by technology
Method used
Image
Examples
Embodiment 1
[0066] like figure 1 As shown, a short-term impact load forecasting method based on two-level decomposition technology includes the following steps:
[0067] S1: Obtain the historical data of impact load and perform average preprocessing on the data;
[0068] It should be noted that the averaging preprocessing is specifically: calculating the average value of the data points included in the impact load history data for every M consecutive data points, and taking the obtained average value as the representative value corresponding to the M data points , where M is a positive integer.
[0069] In this embodiment, the historical impact load of a certain place from June 1, 2010 to December 31, 2011 is obtained, and the time resolution is 5 minutes, that is, there is one data point every 5 minutes. The load prediction time resolution of the present invention is 1 hour. Input the hourly load value of the day before the forecast day into the trained forecasting model, and output t...
PUM
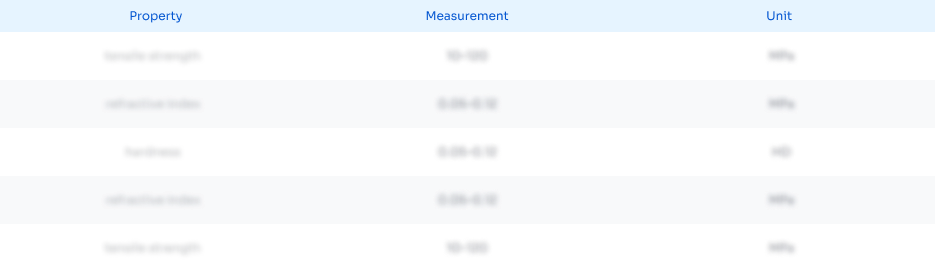
Abstract
Description
Claims
Application Information
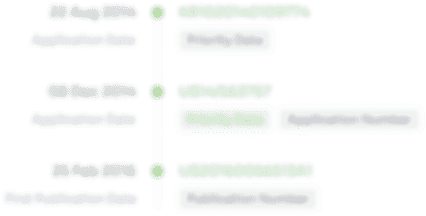
- R&D Engineer
- R&D Manager
- IP Professional
- Industry Leading Data Capabilities
- Powerful AI technology
- Patent DNA Extraction
Browse by: Latest US Patents, China's latest patents, Technical Efficacy Thesaurus, Application Domain, Technology Topic, Popular Technical Reports.
© 2024 PatSnap. All rights reserved.Legal|Privacy policy|Modern Slavery Act Transparency Statement|Sitemap|About US| Contact US: help@patsnap.com