Regional traffic demand prediction method based on convolutional long-term and short-term memory network
A long-short-term memory and traffic demand technology, which is applied in the field of computer data analysis, can solve problems such as low prediction efficiency and insufficient utilization of external influencing factors, and achieve good prediction results, improve generalization ability, and improve prediction accuracy.
- Summary
- Abstract
- Description
- Claims
- Application Information
AI Technical Summary
Problems solved by technology
Method used
Image
Examples
Embodiment Construction
[0061] The present invention will be described in further detail below in conjunction with specific embodiments, but the present invention is not limited to specific embodiments.
[0062] A regional traffic demand forecasting method based on a convolutional long-term short-term memory network, including the training of the network model and the traffic demand forecasting part.
[0063] A method for forecasting regional traffic demand based on convolutional long-short-term memory network, the steps are as follows:
[0064] (1) Training set and test set:
[0065] We evaluate the performance of our proposed network model using the New York taxi dataset (TaxiNY). There are two types of taxis in New York, yellow taxis and green taxis. Yellow taxis pick up passengers mainly in Manhattan, while green taxis operate mostly in the suburbs. The dataset contains GPS trajectories of yellow taxis and green taxis from January 2009 to June 2016. Each track contains the pick-up location an...
PUM
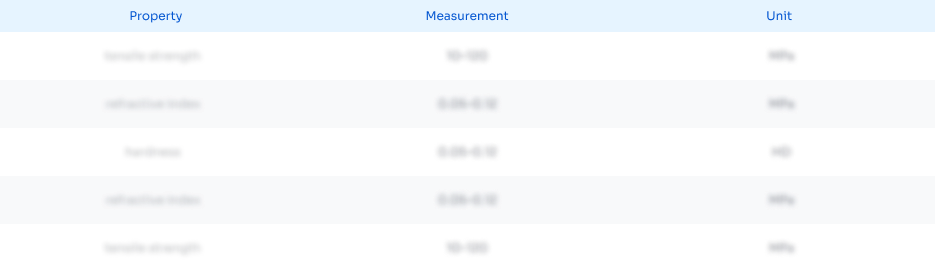
Abstract
Description
Claims
Application Information
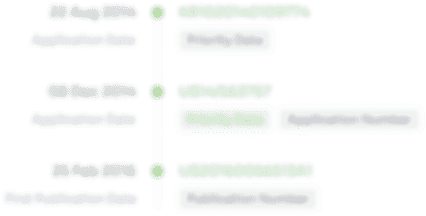
- R&D Engineer
- R&D Manager
- IP Professional
- Industry Leading Data Capabilities
- Powerful AI technology
- Patent DNA Extraction
Browse by: Latest US Patents, China's latest patents, Technical Efficacy Thesaurus, Application Domain, Technology Topic, Popular Technical Reports.
© 2024 PatSnap. All rights reserved.Legal|Privacy policy|Modern Slavery Act Transparency Statement|Sitemap|About US| Contact US: help@patsnap.com