Three-phase rectification control method based on improved adaptive fuzzy neural network
An adaptive fuzzy, three-phase rectification technology, applied in neural learning methods, biological neural network models, neural architectures, etc., can solve the long tail of Gaussian functions, the modeling of type-1 fuzzy systems and the difficulty of minimizing the impact of uncertainty, Stability analysis is difficult, etc.
- Summary
- Abstract
- Description
- Claims
- Application Information
AI Technical Summary
Problems solved by technology
Method used
Image
Examples
Embodiment Construction
[0078] The specific implementation manners of the present invention will be further described in detail below in conjunction with the accompanying drawings and embodiments. The following examples are used to illustrate the present invention, but are not intended to limit the scope of the present invention.
[0079] A three-phase rectification control method based on an improved self-adaptive fuzzy neural network, comprising the following steps:
[0080] Step 1: Through the modeling and analysis of the three-phase rectifier circuit, the voltage and current in the circuit are transformed by synchronous rotating coordinate dq;
[0081] In this embodiment, the three-phase rectification circuit such as figure 1 As shown, the dq axis components are obtained by collecting the electromotive force of the power grid and the AC circuit on the grid side through the synchronous rotation coordinate change. The mathematical expression for the axis is:
[0082]
[0083] Step 2: Use the ...
PUM
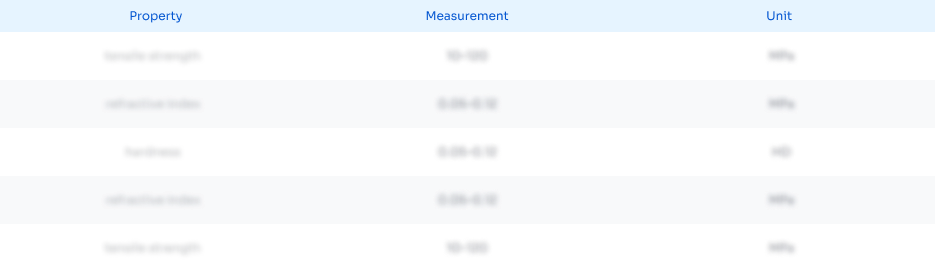
Abstract
Description
Claims
Application Information
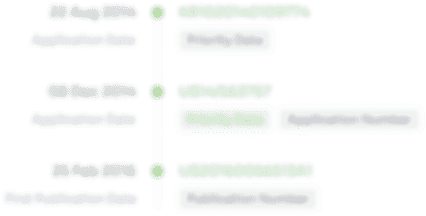
- Generate Ideas
- Intellectual Property
- Life Sciences
- Materials
- Tech Scout
- Unparalleled Data Quality
- Higher Quality Content
- 60% Fewer Hallucinations
Browse by: Latest US Patents, China's latest patents, Technical Efficacy Thesaurus, Application Domain, Technology Topic, Popular Technical Reports.
© 2025 PatSnap. All rights reserved.Legal|Privacy policy|Modern Slavery Act Transparency Statement|Sitemap|About US| Contact US: help@patsnap.com