Clustering result evaluation method and system
A result evaluation and clustering technology, which is applied in the field of clustering result evaluation methods and systems, can solve the problems that the accuracy cannot be directly calculated, the accuracy cannot be directly calculated, and the lack of accuracy of clustering results, etc.
- Summary
- Abstract
- Description
- Claims
- Application Information
AI Technical Summary
Problems solved by technology
Method used
Image
Examples
Embodiment 1
[0030] figure 1 A flow chart of the clustering result evaluation method involved in this embodiment is shown. like figure 1 As shown, the method involved in this embodiment includes:
[0031] Step 102: Extract features of the target. The target may be an object requiring pattern recognition, such as an image.
[0032]Use the recognition algorithm to identify the features of the target. For example, for face recognition, you can use the face recognition algorithm (Shangtang face recognition algorithm) to calculate the face image to be recognized, and calculate the 512-dimensional feature vector (the dimension of the feature vector is related to the algorithm) , and realize eigenvector normalization. The data volume of the feature is M.
[0033] Step 104: Perform clustering processing on the features to obtain clusters. Get the cluster C={C 1 , C 2 ,...,C K}, where K is a positive integer. The clustering results are calculated by clustering algorithms, such as k-means ...
Embodiment 2
[0039] figure 2 A flow chart of the clustering result evaluation method involved in this embodiment is shown. like figure 2 As shown, the present embodiment takes facial image recognition as an example, which includes:
[0040] Step 202: feature extraction of face image.
[0041] The front-end face image acquisition device transmits the face image to the ES (Elasticsearch) database server through the network. Firstly, feature extraction is performed on the face image. The so-called feature extraction refers to the representation of face information through some numbers, and these numbers are the features we want to extract. Common face features are divided into two categories, one is geometric features, and the other is representational features. Geometric features refer to the geometric relationship between facial features such as eyes, nose, and mouth, such as distance, area, and angle. Then use the deep learning neural network algorithm to calculate the final 512-di...
Embodiment 3
[0070] image 3 A schematic diagram of the results of the clustering result evaluation system involved in this embodiment is shown, including: an extraction module 310 , a clustering module 320 , a statistics module 330 , a first calculation module 340 , a second calculation module 350 and a third calculation module 360 .
[0071] The extraction module 310 is used to extract features of the target, and the data volume of the features is M. The clustering module 320 is used to cluster the features to obtain the clusters C={C 1 , C 2 ,...,C K}, wherein K is a positive integer; Statistical module 330, used to count the total number C of pure clusters in the cluster cluster C P , where P is a positive integer and 0≤P≤K; the first calculation module 340 is used to calculate the number of ideal clusters C I ; The second calculation module 350 is used to calculate the data fusion rate correction coefficient n, wherein The third calculation module 360 is used to calculate th...
PUM
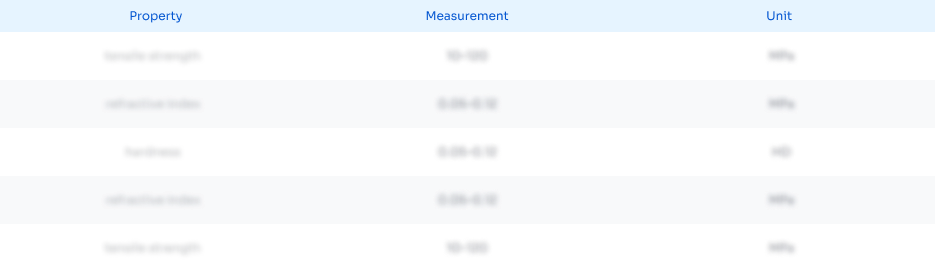
Abstract
Description
Claims
Application Information
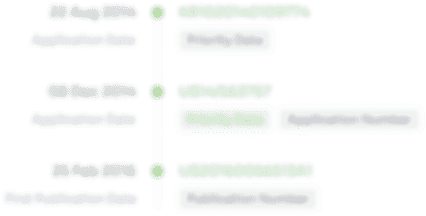
- R&D Engineer
- R&D Manager
- IP Professional
- Industry Leading Data Capabilities
- Powerful AI technology
- Patent DNA Extraction
Browse by: Latest US Patents, China's latest patents, Technical Efficacy Thesaurus, Application Domain, Technology Topic, Popular Technical Reports.
© 2024 PatSnap. All rights reserved.Legal|Privacy policy|Modern Slavery Act Transparency Statement|Sitemap|About US| Contact US: help@patsnap.com