Group activity identification method based on coherence constraint graph long-short-term memory network
A technology of long-term memory and group activity, applied in neural learning methods, character and pattern recognition, biological neural network models, etc., and can solve problems such as exaggerating outlier movements
- Summary
- Abstract
- Description
- Claims
- Application Information
AI Technical Summary
Problems solved by technology
Method used
Image
Examples
Embodiment Construction
[0052] 1. A group activity recognition method based on a coherence-constrained graph long-short-term memory network, including learning the movement state of individuals under the coherence constraints of spatio-temporal context, quantifying the contribution of individual movements to group activities under the coherence constraints of the global context, and using aggregated LSTM to obtain There are four processes of hidden representation of group activities and obtaining probability class vectors of group activities.
[0053] Learning the motion state of an individual under the constraints of spatio-temporal context coherence includes the following steps:
[0054] Step 1. Use a pre-trained convolutional neural network (CNN) model to extract the CNN features of each person in the detected and tracked bounding box. The convolutional neural network used is compatible with AlexNet, VGG, ResNet and GoogLeNet.
[0055] Step 2, add a time confidence gate to the ordinary graph long ...
PUM
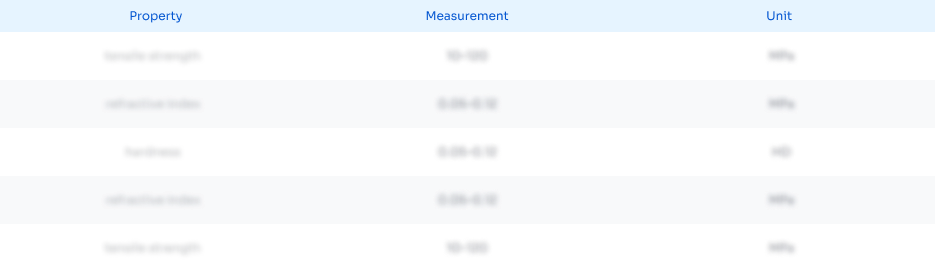
Abstract
Description
Claims
Application Information
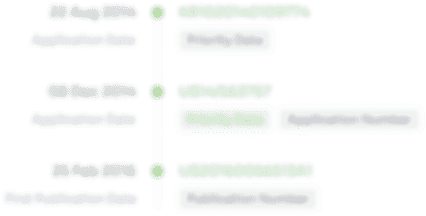
- R&D Engineer
- R&D Manager
- IP Professional
- Industry Leading Data Capabilities
- Powerful AI technology
- Patent DNA Extraction
Browse by: Latest US Patents, China's latest patents, Technical Efficacy Thesaurus, Application Domain, Technology Topic, Popular Technical Reports.
© 2024 PatSnap. All rights reserved.Legal|Privacy policy|Modern Slavery Act Transparency Statement|Sitemap|About US| Contact US: help@patsnap.com