Unsupervised convolutional neural network-based monocular scene depth estimation method
A convolutional neural network and scene depth technology, applied in the field of scene depth estimation, can solve the problems that the depth sensor cannot obtain image depth information, manual data labeling is difficult, and the results are not accurate enough.
- Summary
- Abstract
- Description
- Claims
- Application Information
AI Technical Summary
Problems solved by technology
Method used
Image
Examples
Embodiment Construction
[0069] The present invention will be further described below in conjunction with the accompanying drawings. according to figure 1 The flow chart shown in the figure processes the scene image. First, the camera is used to shoot a video of the scene to be processed, and images of consecutive frames are selected, such as figure 2 As shown, this picture is used as the original image for scene depth estimation in the present invention. According to steps A, B, and C of the present invention, the mapping from the original image to the depth map is realized by using the idea of view synthesis, and the loss function L based on the unsupervised residual convolutional neural network scene depth estimation model is obtained VS As shown in the formula (6), then obtain the feature expression D of the target image according to the step D of the present invention ij (d p , d j ), using the output of these two parts, a scene depth estimation model based on unsupervised conditional ran...
PUM
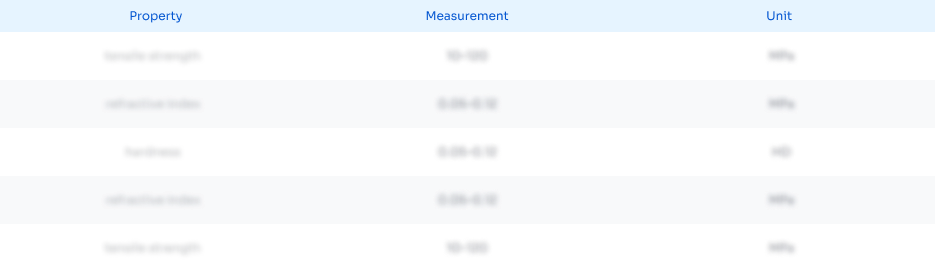
Abstract
Description
Claims
Application Information
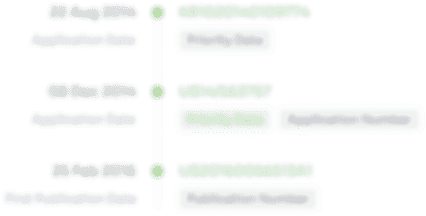
- R&D Engineer
- R&D Manager
- IP Professional
- Industry Leading Data Capabilities
- Powerful AI technology
- Patent DNA Extraction
Browse by: Latest US Patents, China's latest patents, Technical Efficacy Thesaurus, Application Domain, Technology Topic, Popular Technical Reports.
© 2024 PatSnap. All rights reserved.Legal|Privacy policy|Modern Slavery Act Transparency Statement|Sitemap|About US| Contact US: help@patsnap.com