Fault identification method for flexible DC grid based on convolutional neural network
A convolutional neural network, flexible DC technology, applied in fault location, fault detection by conductor type, information technology support system, etc., can solve the problem of insufficient resistance to transition resistance, selectivity and sensitivity can not meet DC fault detection and identification requirements, single-threshold-type judgment method, etc., to achieve the effect of improving the resistance to transition resistance, meeting the quickness, and improving the accuracy
- Summary
- Abstract
- Description
- Claims
- Application Information
AI Technical Summary
Problems solved by technology
Method used
Image
Examples
specific Embodiment 1
[0036] A method for identifying faults in flexible DC power grids based on convolutional neural networks proposed by the present invention, step S1 constructs an initial model of convolutional neural networks for fault identification in flexible DC power grids as follows: figure 1 shown.
[0037] The initial model of the convolutional neural network includes: the first branch structure, the second branch structure, the connection layer, the third convolution layer, the third pooling layer, the random deactivation layer, the first fully connected layer, the second fully connected layer, And the output layer; wherein, the first branch structure includes: the first input layer, the first convolution layer, the first pooling layer; the second branch structure includes: the second input layer, the second convolution layer, the second pooling layer.
[0038] After the first input data is input to the first input layer, it passes through the first convolutional layer and the first p...
specific Embodiment 2
[0043] figure 2 It is a topological structure diagram of a four-terminal flexible DC power grid based on MMC. The topology structure includes: the first converter 1, the second converter 2, the third converter 3, and the fourth converter 4. These four converters all adopt a pseudo-bipolar structure, and the voltage levels are ±200kV. A first line is connected between the first converter 1 and the second converter 2, a second line is connected between the second converter 2 and the third converter 4, and the third converter 4 and the first converter A third line is connected between the four converters 3 , and a fourth line is connected between the fourth converter 3 and the first converter 1 . Both ends of each line are equipped with smoothing reactors and DC circuit breakers near the side of the converter station, and each line adopts a phase-domain frequency-varying model. The fourth line between the first converter 1 and the third converter 3 is used as the test protect...
specific Embodiment 3
[0048] The data of the training data set is normalized and used as the input data of the initial model of the convolutional neural network, and the structure and structure of the initial model of the convolutional neural network are adjusted according to the recognition accuracy and loss function of the output data of the initial model of the convolutional neural network. Parameters, after training and adjustment, when the recognition accuracy reaches 100% and the loss function is less than 0.1, save the model structure and parameters to form a convolutional neural network training model.
[0049] Adjusting the structure of the convolutional neural network initial model specifically includes: adjusting the number of the first convolutional layer, the second convolutional layer and the third convolutional layer, adjusting the first pooling layer, the second pooling layer and the third convolutional layer The number of three-pooling layers, adjusting the number of the first fully...
PUM
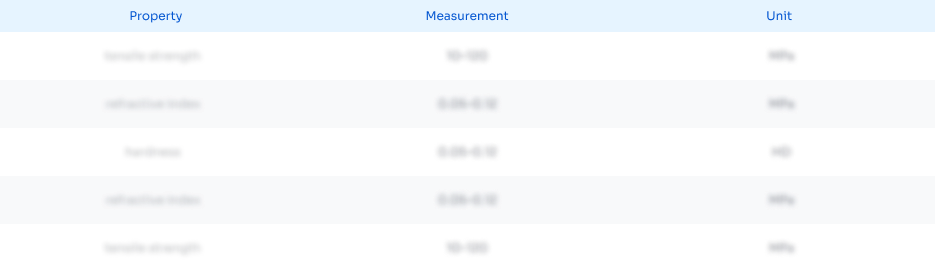
Abstract
Description
Claims
Application Information
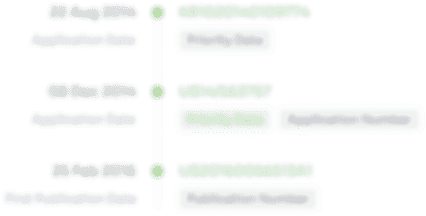
- R&D Engineer
- R&D Manager
- IP Professional
- Industry Leading Data Capabilities
- Powerful AI technology
- Patent DNA Extraction
Browse by: Latest US Patents, China's latest patents, Technical Efficacy Thesaurus, Application Domain, Technology Topic, Popular Technical Reports.
© 2024 PatSnap. All rights reserved.Legal|Privacy policy|Modern Slavery Act Transparency Statement|Sitemap|About US| Contact US: help@patsnap.com