Trend prediction method based on double hidden layer quantum circuit recurrent unit neural network
A cyclic unit, neural network technology, applied in the field of neural networks, can solve the problems of network learning and memory instability, difficult to obtain prediction results, slow learning convergence speed, etc.
- Summary
- Abstract
- Description
- Claims
- Application Information
AI Technical Summary
Problems solved by technology
Method used
Image
Examples
Embodiment Construction
[0137] Such as figure 1 As shown, a trend prediction method based on double-hidden layer quantum circuit recurrent unit neural network includes the following steps:
[0138] S1: Collect the original operating data of the monitored object to construct a permutation entropy set;
[0139] S2: Input the permutation entropy set into the double hidden layer quantum circuit recurrent unit neural network for training and prediction, and obtain the predicted permutation entropy set;
[0140] S3: Calculate the error between the actual permutation entropy and the predicted permutation entropy at each time point, and construct a permutation entropy error set;
[0141] S4: After normalizing the permutation entropy error set, input the double hidden layer quantum circuit recurrent unit neural network for training and prediction, and obtain the predicted normalized permutation entropy error set;
[0142] S5: Denormalize the predicted normalized permutation entropy error set to obtain the f...
PUM
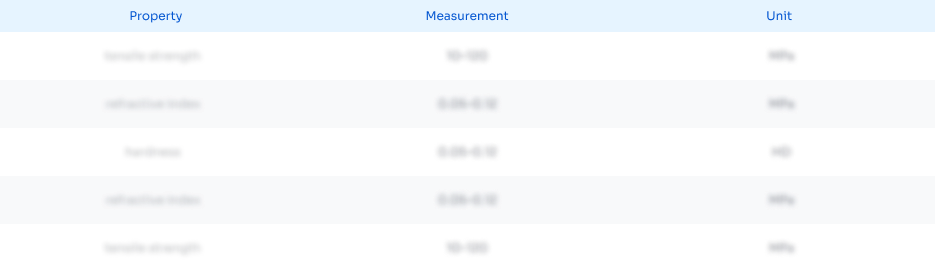
Abstract
Description
Claims
Application Information
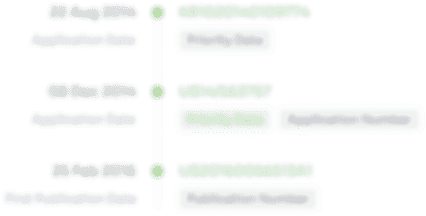
- Generate Ideas
- Intellectual Property
- Life Sciences
- Materials
- Tech Scout
- Unparalleled Data Quality
- Higher Quality Content
- 60% Fewer Hallucinations
Browse by: Latest US Patents, China's latest patents, Technical Efficacy Thesaurus, Application Domain, Technology Topic, Popular Technical Reports.
© 2025 PatSnap. All rights reserved.Legal|Privacy policy|Modern Slavery Act Transparency Statement|Sitemap|About US| Contact US: help@patsnap.com