Multivariate monitoring time series regression prediction method for energy chemical production system
A time series, chemical production technology, applied in the direction of design optimization/simulation, etc., can solve the problems of multivariate monitoring time series without auxiliary monitoring time series selection method, non-linearity, and unsatisfactory results obtained.
- Summary
- Abstract
- Description
- Claims
- Application Information
AI Technical Summary
Problems solved by technology
Method used
Image
Examples
Embodiment
[0116] Example: Using the actual monitoring data of the compressor unit in the production system of an energy and chemical enterprise, the regression prediction method based on the improved DESN multivariate monitoring time series is described in detail.
[0117] Select the historical data of 12 monitoring time series in the compressor unit, and the relevant information of each monitoring time series is shown in the following table:
[0118] Table 1 Monitoring time series information table
[0119]
[0120]
[0121] The actual monitoring data of the above monitoring points within 1 day is exported from the distributed control system of the compressor unit as sample data, the sampling interval is one minute, and the total amount of data is 1440. According to the research plan of the present invention, the monitoring data sequence of the No. 6 point is used as the monitoring time series of the target to be predicted, and the coupling relationship between the target monitor...
PUM
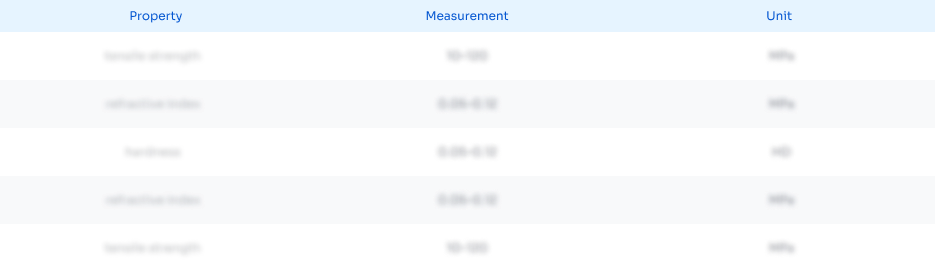
Abstract
Description
Claims
Application Information
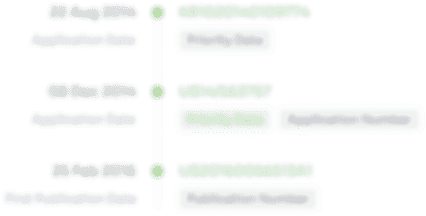
- Generate Ideas
- Intellectual Property
- Life Sciences
- Materials
- Tech Scout
- Unparalleled Data Quality
- Higher Quality Content
- 60% Fewer Hallucinations
Browse by: Latest US Patents, China's latest patents, Technical Efficacy Thesaurus, Application Domain, Technology Topic, Popular Technical Reports.
© 2025 PatSnap. All rights reserved.Legal|Privacy policy|Modern Slavery Act Transparency Statement|Sitemap|About US| Contact US: help@patsnap.com