Lightweight deep network image target detection method suitable for Raspberry Pi
A deep network and target detection technology, which is applied in the field of deep learning and target detection, can solve the problems of reducing network parameters and calculation amount, degrading detection accuracy performance, and difficulty of deep detection model, so as to improve regression rate and positioning accuracy, hardware memory and the effect of reducing computing power requirements and expanding the receptive field
- Summary
- Abstract
- Description
- Claims
- Application Information
AI Technical Summary
Problems solved by technology
Method used
Image
Examples
Embodiment Construction
[0044]In order to make the purpose of the method of the present invention, technical solutions and advantages more clear, the present invention is explained below in conjunction with the accompanying drawings and examples, and is not intended to limit the present invention:
[0045] Step 1. Collect images containing the target to be detected, and preprocess the collected images for network training.
[0046] Select the target category to be detected, then collect a large number of images containing these categories of targets, and mark the target, that is, mark its bounding box and category information for each target to be detected that appears in each image;
[0047] When the number of collected images is small, use the existing images to perform data enhancement operations. Create more images by flipping, translating, rotating or adding noise, so that the trained neural network has better results;
[0048] Uniformly convert the image resolution to 224*224 to fit the input ...
PUM
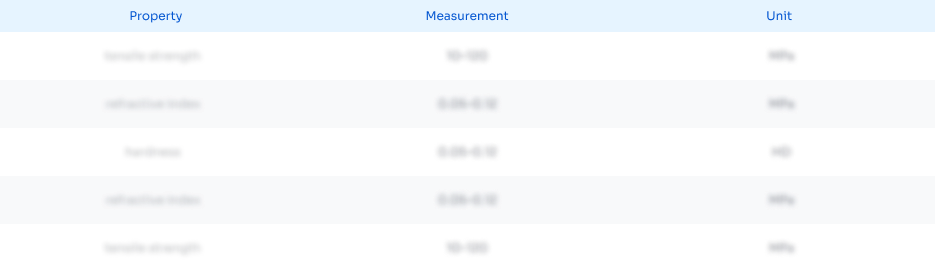
Abstract
Description
Claims
Application Information
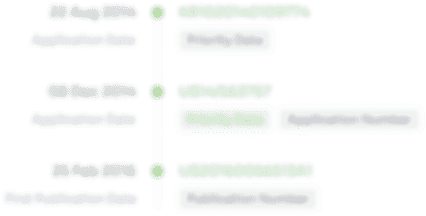
- Generate Ideas
- Intellectual Property
- Life Sciences
- Materials
- Tech Scout
- Unparalleled Data Quality
- Higher Quality Content
- 60% Fewer Hallucinations
Browse by: Latest US Patents, China's latest patents, Technical Efficacy Thesaurus, Application Domain, Technology Topic, Popular Technical Reports.
© 2025 PatSnap. All rights reserved.Legal|Privacy policy|Modern Slavery Act Transparency Statement|Sitemap|About US| Contact US: help@patsnap.com