Rolling bearing fault diagnosis method based on two-way memory cycle neural network
A cyclic neural network and fault diagnosis technology, applied in neural learning methods, biological neural network models, neural architectures, etc., can solve the problem that the fault diagnosis model does not consider the unsteady characteristics of bearing data acquisition, the data logic structure is single, and the fault cannot be judged. type, etc.
- Summary
- Abstract
- Description
- Claims
- Application Information
AI Technical Summary
Problems solved by technology
Method used
Image
Examples
Embodiment Construction
[0029] The present invention will be further described below.
[0030] As shown in the figure, the concrete steps of the present invention are:
[0031]A. Obtain program data samples: install acceleration sensors at different vibration detection points of the bearing, each acceleration sensor collects vibration acceleration data in the horizontal and vertical directions of the bearing, the sampling frequency is 48000Hz, and then standardize the vibration acceleration data Preprocessing , so that the collected data conforms to the standard normal distribution, and then a signal with a length of 2000 data points is intercepted from the standardized processed data as a program data sample;
[0032] B. Use feature extraction algorithm to decompose program data samples: use time-frequency domain feature extraction algorithm (such as wavelet packet transform method) to decompose program data samples into 9 layers, and use the vibration signal frequency band energy of the 9th layer a...
PUM
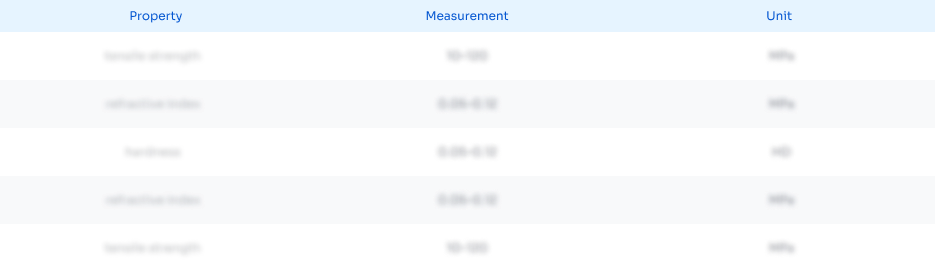
Abstract
Description
Claims
Application Information
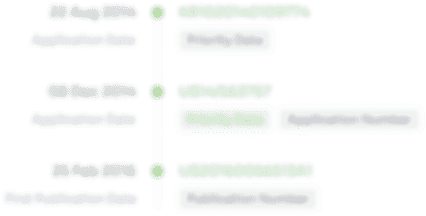
- R&D
- Intellectual Property
- Life Sciences
- Materials
- Tech Scout
- Unparalleled Data Quality
- Higher Quality Content
- 60% Fewer Hallucinations
Browse by: Latest US Patents, China's latest patents, Technical Efficacy Thesaurus, Application Domain, Technology Topic, Popular Technical Reports.
© 2025 PatSnap. All rights reserved.Legal|Privacy policy|Modern Slavery Act Transparency Statement|Sitemap|About US| Contact US: help@patsnap.com