Pulmonary nodule image classification method when uncertain data is contained in data set
A technology for determining data and data concentration, applied in the field of computer vision, can solve problems such as difficult training data, inconsistent labels, and heavy workload
- Summary
- Abstract
- Description
- Claims
- Application Information
AI Technical Summary
Problems solved by technology
Method used
Image
Examples
Embodiment Construction
[0044] The present invention will be further described below in conjunction with the accompanying drawings and specific embodiments.
[0045] Such as figure 1 As shown, it is a flow chart of the pulmonary nodule image classification method when the data set of the present invention contains uncertain data. The lung nodule image classification method when a data set of the present invention contains uncertain data is characterized in that it comprises the following steps:
[0046] Step 1: organize the data set: collect N CT images of lung nodules to form an image set I={I 1 , I 2 ,...,I n ,...,I N}, the lung nodules are divided into three types: benign, malignant and indeterminate; the expert method is used to classify each lung nodule CT image, and the nth lung nodule CT image I is obtained n The category is e n ; Each piece of pulmonary nodule CT image is preprocessed to obtain a pulmonary nodule CT image data set D; the preprocessing includes converting the pulmonary n...
PUM
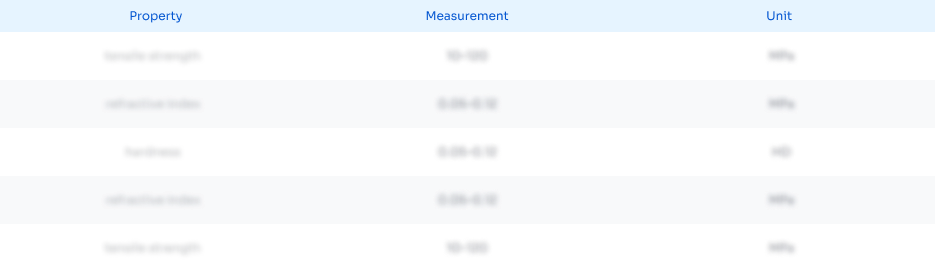
Abstract
Description
Claims
Application Information
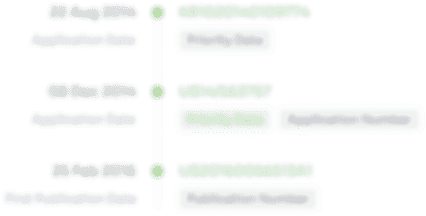
- R&D Engineer
- R&D Manager
- IP Professional
- Industry Leading Data Capabilities
- Powerful AI technology
- Patent DNA Extraction
Browse by: Latest US Patents, China's latest patents, Technical Efficacy Thesaurus, Application Domain, Technology Topic, Popular Technical Reports.
© 2024 PatSnap. All rights reserved.Legal|Privacy policy|Modern Slavery Act Transparency Statement|Sitemap|About US| Contact US: help@patsnap.com