A Multi-model Interval Prediction Method for Photovoltaic Power
A prediction method and multi-model technology, applied in prediction, neural learning method, biological neural network model, etc., can solve the problems of different distribution characteristics, engineering application constraints, difficulty in accurate prediction, etc., to achieve high fitting accuracy and easy implementation. , the effect of good prediction accuracy
- Summary
- Abstract
- Description
- Claims
- Application Information
AI Technical Summary
Problems solved by technology
Method used
Image
Examples
Embodiment Construction
[0051] In order to enable those skilled in the art to better understand the present invention, the technical solution of the present invention will be further described below in conjunction with the accompanying drawings and embodiments.
[0052] A photovoltaic power multi-model interval prediction method based on extreme learning machine and kernel density estimation, the flow chart is as follows figure 1 As shown, the specific steps are as follows:
[0053] S1. Collect historical operating data of photovoltaic power plants, historical environmental data and numerical weather forecast data.
[0054] The historical operation data includes the historical power data of the photovoltaic power station, the historical environmental data includes the historical irradiance, ambient temperature, humidity, and wind speed data corresponding to the photovoltaic power station, and the numerical weather forecast data includes the numerical weather forecast irradiance and ambient temperature ...
PUM
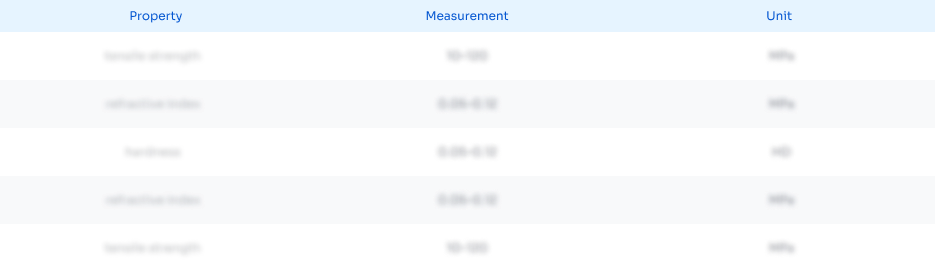
Abstract
Description
Claims
Application Information
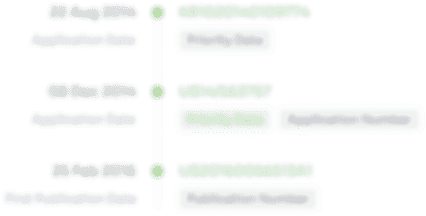
- R&D Engineer
- R&D Manager
- IP Professional
- Industry Leading Data Capabilities
- Powerful AI technology
- Patent DNA Extraction
Browse by: Latest US Patents, China's latest patents, Technical Efficacy Thesaurus, Application Domain, Technology Topic, Popular Technical Reports.
© 2024 PatSnap. All rights reserved.Legal|Privacy policy|Modern Slavery Act Transparency Statement|Sitemap|About US| Contact US: help@patsnap.com