Semi-supervised image retrieval method based on self-coding network and robust kernel hashing
A self-encoding network and image retrieval technology, which is applied in the field of large-scale image retrieval, can solve problems such as poor robustness and large amount of calculation, and achieve the effect of improving robustness and reducing computational complexity
- Summary
- Abstract
- Description
- Claims
- Application Information
AI Technical Summary
Problems solved by technology
Method used
Image
Examples
Embodiment Construction
[0044] The present invention first uses a self-encoding network structure to extract deep image features. The process of training the self-encoding network is to sequentially input the images in the public image data set into the network, and use the random gradient descent method to update the network parameters by calculating the loss of the network. When the training reaches the specified number of times Or the training is completed when the loss function becomes stable. After the training is completed, the network structure of the encoding stage is selected as the image depth feature extraction network. Since the images in the retrieval library are relatively fixed, in order to speed up the retrieval, all the images in the retrieval library can be pre-generated with corresponding depth features.
[0045] The self-encoding network is an encoding-decoding structure. In the encoding stage, the input feature map of each layer is represented by a three-dimensional array [h,w,d], wh...
PUM
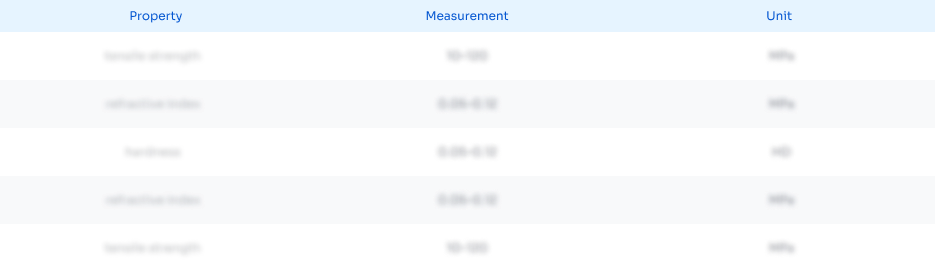
Abstract
Description
Claims
Application Information
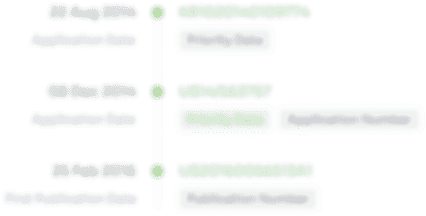
- R&D Engineer
- R&D Manager
- IP Professional
- Industry Leading Data Capabilities
- Powerful AI technology
- Patent DNA Extraction
Browse by: Latest US Patents, China's latest patents, Technical Efficacy Thesaurus, Application Domain, Technology Topic, Popular Technical Reports.
© 2024 PatSnap. All rights reserved.Legal|Privacy policy|Modern Slavery Act Transparency Statement|Sitemap|About US| Contact US: help@patsnap.com