A Network Optimal Tracking Control Method Based on Off-Policy q-Learning
A tracking control and network control system technology, applied in the field of network optimal tracking control based on off-policy Q-learning
- Summary
- Abstract
- Description
- Claims
- Application Information
AI Technical Summary
Problems solved by technology
Method used
Image
Examples
Embodiment Construction
[0026] The present invention will be described in detail below in conjunction with examples.
[0027] 1. Optimization with packet loss compensation
[0028] The invention introduces the linear quadratic tracking (LQT) problem and the model of network induced packet loss, and expounds the quadratic tracking problem of the network control system with data packet loss.
[0029] Consider the following linear discrete system
[0030]
[0031] in, is the state of the controlled object, and is dimension, is the charged input, for dimension, is the controlled output, for dimension. respectively and dimension.
[0032] The reference signal is as follows
[0033] (2)
[0034] in, is the reference input, for dimension, for dimension. In this tracking question, wanting the output in system (1) track reference input .
[0035] make , from formula (1) and formula (2), get the following augmented system
[0036] (3)
[0037] in, .
[0038]...
PUM
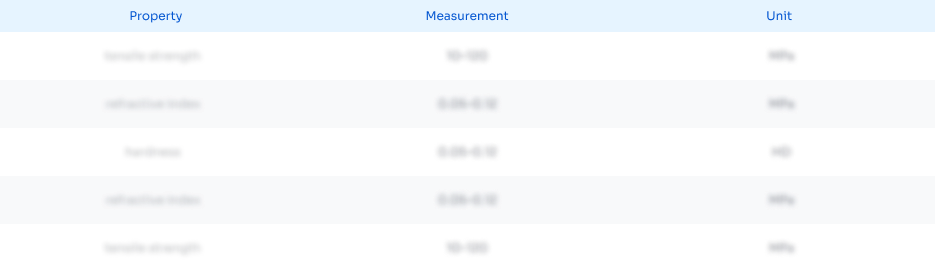
Abstract
Description
Claims
Application Information
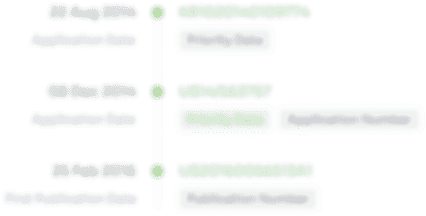
- R&D Engineer
- R&D Manager
- IP Professional
- Industry Leading Data Capabilities
- Powerful AI technology
- Patent DNA Extraction
Browse by: Latest US Patents, China's latest patents, Technical Efficacy Thesaurus, Application Domain, Technology Topic, Popular Technical Reports.
© 2024 PatSnap. All rights reserved.Legal|Privacy policy|Modern Slavery Act Transparency Statement|Sitemap|About US| Contact US: help@patsnap.com