Multi-view multi-mark classification method based on view category characteristic learning
A feature learning and classification method technology, applied in the direction of instruments, character and pattern recognition, computer components, etc., can solve the problems of not considering the contribution degree and the inaccurate weight of the perspective contribution degree, etc.
- Summary
- Abstract
- Description
- Claims
- Application Information
AI Technical Summary
Problems solved by technology
Method used
Image
Examples
Embodiment Construction
[0047] In order to make the purpose and technical solutions of the embodiments of the present invention clearer, the technical solutions of the embodiments of the present invention will be described clearly and completely in conjunction with the accompanying drawings of the embodiments of the present invention. Obviously, the described embodiments are part of the embodiments of the present invention, rather than all of the embodiments. Based on the described embodiments of the present invention, all other embodiments obtained by a person of ordinary skill in the art without creative work shall fall within the protection scope of the present invention.
[0048] Such as figure 1 As shown, a multi-view and multi-label classification method based on perspective category feature learning includes the following steps:
[0049] S1. Obtain training data, classify the training data, and establish a class label matrix;
[0050] S2. After constructing the category labeling, each perspective ...
PUM
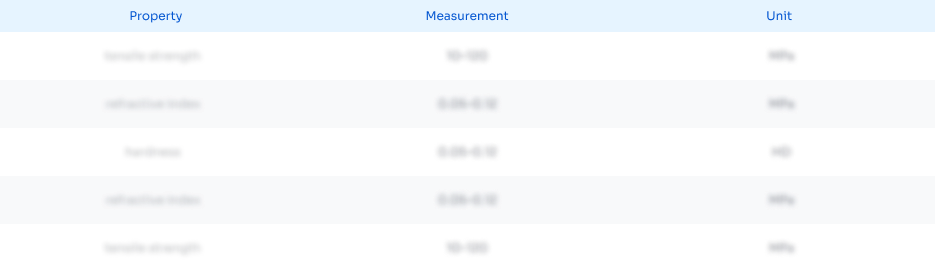
Abstract
Description
Claims
Application Information
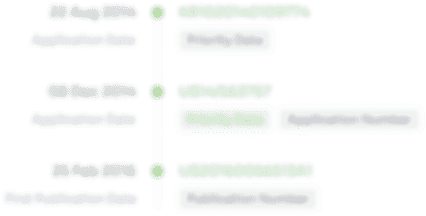
- R&D Engineer
- R&D Manager
- IP Professional
- Industry Leading Data Capabilities
- Powerful AI technology
- Patent DNA Extraction
Browse by: Latest US Patents, China's latest patents, Technical Efficacy Thesaurus, Application Domain, Technology Topic, Popular Technical Reports.
© 2024 PatSnap. All rights reserved.Legal|Privacy policy|Modern Slavery Act Transparency Statement|Sitemap|About US| Contact US: help@patsnap.com