A processing method for high-order tensor data
A processing method and high-level technology, applied in the field of data processing, can solve the problems of complex feature extraction of test samples, and achieve the effect of improving image processing speed and simplifying a large amount of redundant information.
- Summary
- Abstract
- Description
- Claims
- Application Information
AI Technical Summary
Problems solved by technology
Method used
Image
Examples
Embodiment Construction
[0011] like figure 1 As shown, this processing method for high-order tensor data decomposes high-order tensor data into three parts: shared subspace components, individual subspace components, and noise parts; shared subspace components and individual subspace components respectively The high-order tensor data is expressed as a linear combination of a set of tensor bases and vector coefficients; the variational EM method is used to solve the base tensor and vector coefficients; a classifier is designed to classify the samples to be tested by comparing the marginal distribution of samples.
[0012] Compared with the traditional linear discriminant method, the present invention directly acts on the tensor data and extracts common features and individual features from two spaces, and constructs a tensor data in each space by utilizing the structural characteristics of the tensor The representation of vector data can be used to extract the discriminative features of tensor samples...
PUM
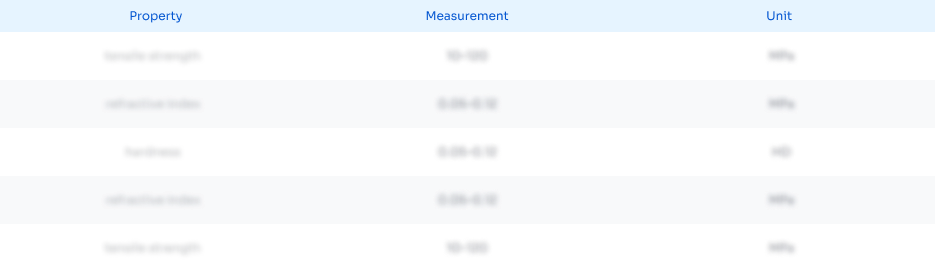
Abstract
Description
Claims
Application Information
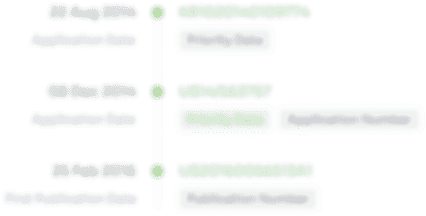
- R&D Engineer
- R&D Manager
- IP Professional
- Industry Leading Data Capabilities
- Powerful AI technology
- Patent DNA Extraction
Browse by: Latest US Patents, China's latest patents, Technical Efficacy Thesaurus, Application Domain, Technology Topic.
© 2024 PatSnap. All rights reserved.Legal|Privacy policy|Modern Slavery Act Transparency Statement|Sitemap