Learning-based low-delay task scheduling method in edge computing network
An edge computing and task scheduling technology, applied in the field of mobile computing, which can solve problems such as difficult design, heuristic algorithm environment changes, etc.
- Summary
- Abstract
- Description
- Claims
- Application Information
AI Technical Summary
Problems solved by technology
Method used
Image
Examples
Embodiment 1
[0032] This embodiment discloses a learning-based low-latency task scheduling method in an edge computing network. Mobile smart terminals held by multiple users communicate with a multi-resource server (EC server) cluster in the edge computing network through a wireless access point. Connection, only keep the system state of N tasks arriving at each time, and put the task information other than N in the backlog part, only count the number of tasks, and schedule N tasks at each time step, allowing the agent Agent Execute multiple actions a at each time step. At each time step t, time is frozen until an invalid action is selected or an inappropriate task is attempted to be scheduled. The time will not proceed. The cluster image moves one step, each time a The time step is equivalent to the agent making an effective decision, and then the agent observes the state transition, that is, the task is scheduled to the appropriate position in the cluster image; the reward is set at each ...
Embodiment 2
[0053] Such as figure 1 shown. Mobile smart terminals held by multiple users are connected to a server (EC server) cluster in the edge computing network through a wireless access point, and the EC server cluster is a multi-resource cluster. Tasks dynamically arrive at the edge server cluster online, and once a task is scheduled, it cannot be preempted. We assume an edge server cluster with three types of resources (CPU, memory, I / O), tasks generated by mobile smart terminals arrive at the edge network server cluster online at discrete time steps, and select a or multiple tasks for scheduling. The resource requirements of each task are assumed to be known upon arrival. For a smart mobile terminal i, the task it generates is denoted as A i =(d i ,c i ,r i ), where d i , representing task A i The data size of c i Indicates the completion of task A i The total number of CPU cycles required, r i Indicates task A i Required IO resources
[0054] This paper hopes to min...
PUM
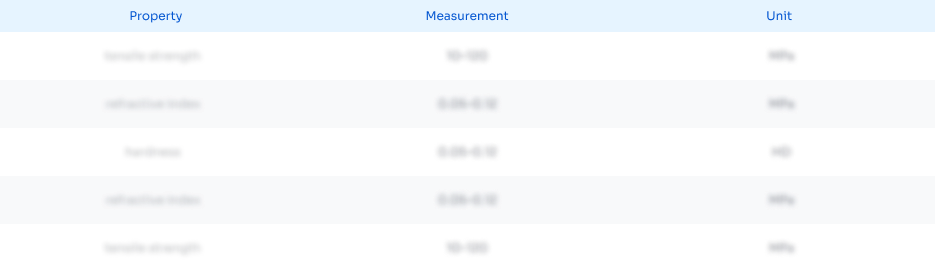
Abstract
Description
Claims
Application Information
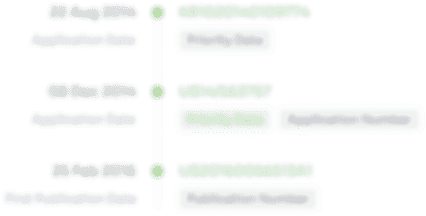
- R&D Engineer
- R&D Manager
- IP Professional
- Industry Leading Data Capabilities
- Powerful AI technology
- Patent DNA Extraction
Browse by: Latest US Patents, China's latest patents, Technical Efficacy Thesaurus, Application Domain, Technology Topic, Popular Technical Reports.
© 2024 PatSnap. All rights reserved.Legal|Privacy policy|Modern Slavery Act Transparency Statement|Sitemap|About US| Contact US: help@patsnap.com