An urban rail short-time passenger flow prediction method based on modal decomposition and deep learning
A technology of deep learning and modal decomposition, applied in forecasting, biological neural network models, instruments, etc., can solve the problems of timing, non-stationarity and nonlinearity of short-term passenger flow data, and overcome the defects of gradient explosion , Maintain information persistence and improve stability
- Summary
- Abstract
- Description
- Claims
- Application Information
AI Technical Summary
Problems solved by technology
Method used
Image
Examples
Embodiment Construction
[0021] A short-term passenger flow prediction method for urban rail based on modal decomposition and deep learning, such as figure 1 As shown, it mainly includes the CEEMDAN decomposition stage, the Fine-to-Coarse reconstruction stage, and the GRU prediction stage. The specific content is as follows:
[0022] 1. CEEMDAN decomposition stage
[0023] At this stage, the passenger flow data of urban rail transit is regarded as a piece of data composed of continuous smooth signal and abnormal noise. Use CEEMDAN to decompose and process the original passenger flow data. For a given data x(t), EMD can decompose the data to obtain a set of intrinsic mode functions IMF from high to low frequencies i (t), (i=1, K, I), I is the number of decomposition, high frequency (short period) IMF i (t) represents the high time-varying nature in the original data, low frequency (long period) IMF i (t) represents the long-period characteristics in the original data. The specific process of EMD d...
PUM
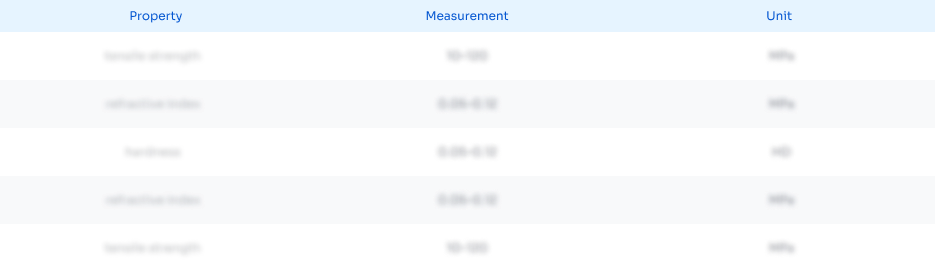
Abstract
Description
Claims
Application Information
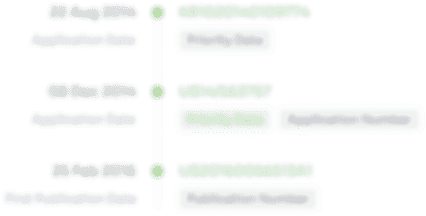
- R&D Engineer
- R&D Manager
- IP Professional
- Industry Leading Data Capabilities
- Powerful AI technology
- Patent DNA Extraction
Browse by: Latest US Patents, China's latest patents, Technical Efficacy Thesaurus, Application Domain, Technology Topic, Popular Technical Reports.
© 2024 PatSnap. All rights reserved.Legal|Privacy policy|Modern Slavery Act Transparency Statement|Sitemap|About US| Contact US: help@patsnap.com