A quantum particle swarm multi-objective optimization method
A multi-objective optimization and multi-objective technology, applied in the field of multi-objective optimization of quantum particle swarms, can solve problems such as accuracy decline, achieve uniform distribution, widen the search range, and avoid easy convergence to boundary solutions.
- Summary
- Abstract
- Description
- Claims
- Application Information
AI Technical Summary
Problems solved by technology
Method used
Image
Examples
Embodiment
[0056] The present invention discloses a multi-objective optimization method for quantum particle swarms, which includes the following steps:
[0057] S1. Establish a simplified quantum double potential well model based on double delta potential well;
[0058] S2, establishing a particle position update model based on the double potential well model;
[0059] S3. Construct a shared learning strategy for particles;
[0060] S4. Construct a multi-objective optimization algorithm for quantum particle swarms.
[0061] In step S1, the quantum double potential well model formula is:
[0062]
[0063] In formula (1), ψ(Y 1 ) And ψ(Y 2 ) Are the inner delta potential well wave function and the outer delta potential well wave function respectively; β is the potential well distance factor, and the value of β is determined according to the potential well distance:
[0064]
[0065] In formula (2), d is the distance of the potential well; Is the normalized value, d 1 And d 2 They are the critical d...
PUM
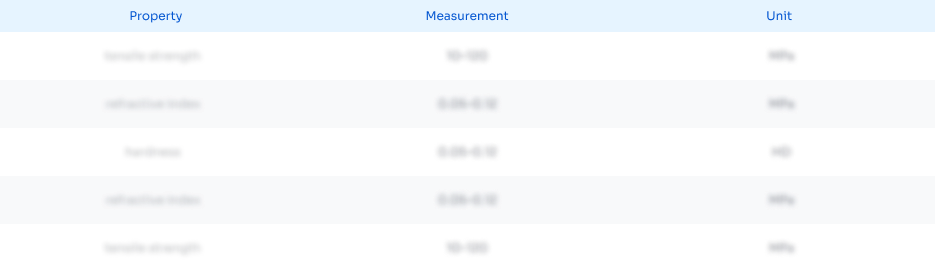
Abstract
Description
Claims
Application Information
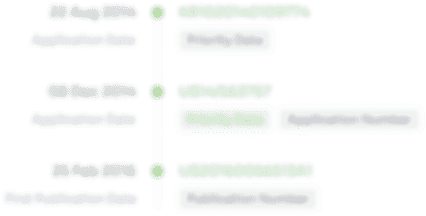
- R&D Engineer
- R&D Manager
- IP Professional
- Industry Leading Data Capabilities
- Powerful AI technology
- Patent DNA Extraction
Browse by: Latest US Patents, China's latest patents, Technical Efficacy Thesaurus, Application Domain, Technology Topic.
© 2024 PatSnap. All rights reserved.Legal|Privacy policy|Modern Slavery Act Transparency Statement|Sitemap