Blast heap ore rock particle image segmentation method
An image segmentation, ore-rock technology, applied in the field of image processing, can solve problems such as serious adhesion of ore-rock particles, inaccurate contour extraction, complex parameter adjustment, etc., to avoid over-segmentation and under-segmentation, good light adaptive performance, and segmentation high precision effect
- Summary
- Abstract
- Description
- Claims
- Application Information
AI Technical Summary
Problems solved by technology
Method used
Image
Examples
Embodiment 1
[0048] Such as Figure 4 As shown, the invention provides a method for image segmentation of explosive pile ore rock particles, specifically comprising the following steps:
[0049] As shown in 1, obtaining the pre-trained first convolutional neural network and the second convolutional neural network includes:
[0050] A1, constructing the first convolutional neural network and the second convolutional neural network;
[0051] Such as figure 2 As shown, for example, the first convolutional neural network is a U-Net convolutional neural network whose input image size is 48×48; as image 3 The second convolutional neural network shown is a U-Net convolutional neural network with an input image size of 480×480. The U-Net convolutional network includes a 3×3 convolutional layer, a maximum pooling layer, and a 2×2 upsampling volume Convolutional Layers and 1×1 Convolutional Layers
[0052] The U-Net convolutional network adopted in the present invention is based on a deformati...
Embodiment 2
[0077] In the present embodiment, for any stope acquisition format is an image of explosive pile ore rock particles in RGB, such as Figure 5 As shown; it includes images with uneven illumination, complex surface texture of ore rock particles, and insufficient contrast. In addition, since the original image is in RGB format, this will increase the computational complexity in network training, so it needs to be equal to the original two-dimensional image Perform grayscale and bilateral filtering.
[0078] For the first time, put it into the first convolutional network model with an input size of 48×48 pre-training to get the first probability map, such as Figure 6 Shown; Use OpenCV to extract the contour and get the first contour map and calculate the S value of the contour map.
[0079] Generate the nth contour map for any nth probability map, use the nth contour map to modify the first probability map to obtain the nth probability correction map to correct the probability m...
PUM
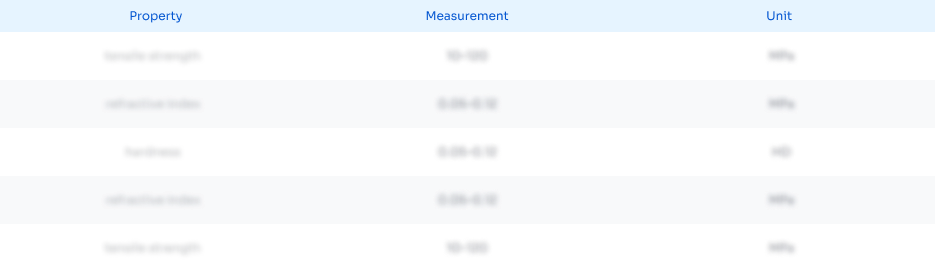
Abstract
Description
Claims
Application Information
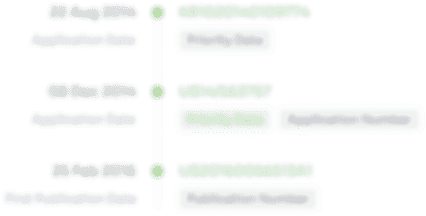
- R&D Engineer
- R&D Manager
- IP Professional
- Industry Leading Data Capabilities
- Powerful AI technology
- Patent DNA Extraction
Browse by: Latest US Patents, China's latest patents, Technical Efficacy Thesaurus, Application Domain, Technology Topic.
© 2024 PatSnap. All rights reserved.Legal|Privacy policy|Modern Slavery Act Transparency Statement|Sitemap