Floor defect automatic detection method based on computer vision and deep learning
A computer vision and deep learning technology, applied in computing, image data processing, instruments, etc., can solve problems such as difficulty in improving accuracy and speed at the same time, detection effect easily affected by the environment, and poor algorithm adaptability.
- Summary
- Abstract
- Description
- Claims
- Application Information
AI Technical Summary
Problems solved by technology
Method used
Image
Examples
Embodiment
[0102] figure 1 A schematic flow chart of the method for automatic detection of floor image defects based on computer vision and deep learning in this embodiment is shown.
[0103] The embodiment adopts the actual image of the wall of a residential area, the resolution is 7952*5304, and the file size is generally about 30M. Initially, 70 defect images and 169 defect targets were obtained. As shown in the figure, the green frame is a defect.
[0104] First, according to step 1, pre-train the defect detection model InceptionV2-SSD (single shot multibox detector) on the COCO training set. During the training process, the highest average precision index (COCO mAP) of the model is 24, freeze the model of this node, and obtain the model migration The learned initialization parameters.
[0105] According to step 2, the expansion coefficient a is set to 0.3, and the corrected sample of the initial sample set is as follows Figure 2 to Figure 5 shown (both original and enlarged). ...
PUM
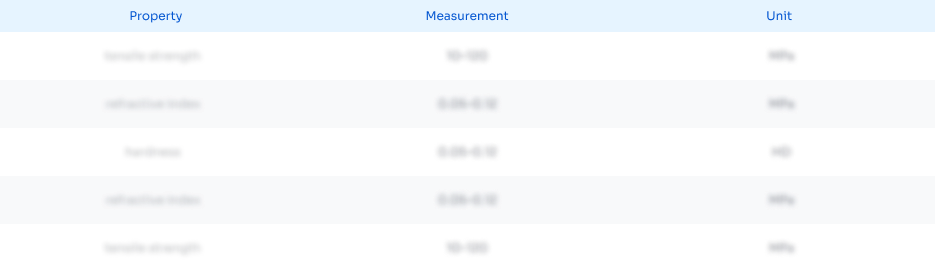
Abstract
Description
Claims
Application Information
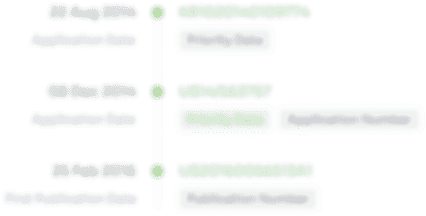
- R&D Engineer
- R&D Manager
- IP Professional
- Industry Leading Data Capabilities
- Powerful AI technology
- Patent DNA Extraction
Browse by: Latest US Patents, China's latest patents, Technical Efficacy Thesaurus, Application Domain, Technology Topic, Popular Technical Reports.
© 2024 PatSnap. All rights reserved.Legal|Privacy policy|Modern Slavery Act Transparency Statement|Sitemap|About US| Contact US: help@patsnap.com