Computer-aided system and method for detecting mammary molybdenum target lumps through data driving
A computer-aided, data-driven technology, applied in computer parts, computing, instruments, etc., can solve the problems of lack of medical principle support for training models, low model recognition rate, large data requirements, etc., to improve generalization ability and recognition rate. , the effect of reducing training data and simple model structure
- Summary
- Abstract
- Description
- Claims
- Application Information
AI Technical Summary
Problems solved by technology
Method used
Image
Examples
Embodiment 1
[0135] A data-driven computer-aided system for mammography mass detection comprising
[0136] The image processor is used to label and classify the collected clinical images, and the images are divided into a training set, a verification set and a test set;
[0137] ROI extractor for extracting the mass region in the processed image;
[0138] Data-driven model, used to build and train extractors that are good at extracting tumor area shape, edge texture, and density features;
[0139] The comprehensive recognition model is used to construct the CNN network to extract the overall features of the tumor, and then fuse the features obtained by the extractor corresponding to the data-driven model with the overall features of the tumor, and use the training set and verification set for training and verification to obtain the best Comprehensive recognition model, input the test set data into the best comprehensive recognition model for recognition, and obtain the detection results. ...
Embodiment 2
[0149] Based on Example 1, the data-driven model includes a shape feature extractor, an edge texture feature extractor, and a density feature extractor, the details of which are as follows:
[0150] The shape feature extractor includes an edge extraction unit for extracting the edge of the tumor area, an edge connection unit for connecting the extracted edges to obtain a closed edge, a filling unit for filling the closed edge with a solid color to obtain the shape of the tumor, and a unit for using the above-mentioned unit The processed training set and verification set are used to train and verify the constructed CNN network, and obtain a shape feature CNN model that can obtain the best shape features and is good at distinguishing three types of shapes: circular, oval, and irregular;
[0151] The shape feature extractor training includes the following steps:
[0152] Step a1: extracting edges in the mass region;
[0153] Step b1: connect the extracted edges to obtain closed ...
PUM
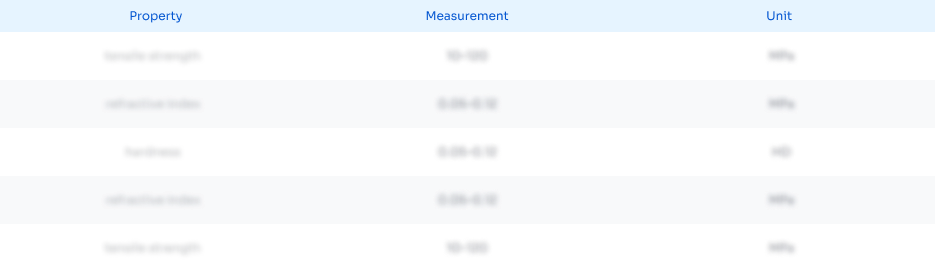
Abstract
Description
Claims
Application Information
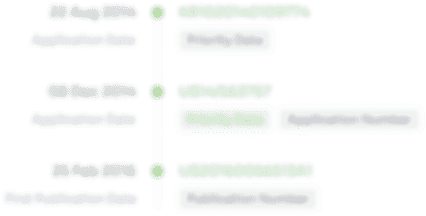
- R&D
- Intellectual Property
- Life Sciences
- Materials
- Tech Scout
- Unparalleled Data Quality
- Higher Quality Content
- 60% Fewer Hallucinations
Browse by: Latest US Patents, China's latest patents, Technical Efficacy Thesaurus, Application Domain, Technology Topic, Popular Technical Reports.
© 2025 PatSnap. All rights reserved.Legal|Privacy policy|Modern Slavery Act Transparency Statement|Sitemap|About US| Contact US: help@patsnap.com