Depth wavelet extreme learning machine-based multi-level inverter fault diagnosis method
A multi-level inverter and extreme learning machine technology, applied in neural learning methods, measuring electrical variables, instruments, etc., can solve problems such as slow training speed, low degree of automation, and large manual intervention, and achieve easy implementation and operation. simple effect
- Summary
- Abstract
- Description
- Claims
- Application Information
AI Technical Summary
Problems solved by technology
Method used
Image
Examples
Embodiment Construction
[0047] In order to make the object, technical solution and advantages of the present invention clearer, the present invention will be further described in detail below in conjunction with the accompanying drawings and embodiments. It should be understood that the specific embodiments described here are only used to explain the present invention, not to limit the present invention.
[0048] like figure 1 Shown is a schematic flow chart of a multilevel inverter fault diagnosis method based on a deep wavelet extreme learning machine of the present invention; a multilevel inverter fault diagnosis method based on a deep wavelet extreme learning machine comprises the following steps:
[0049] A. Use the voltage sensor to collect voltage fault signals on the AC side of the inverter under various working conditions;
[0050] B. The three-phase AC voltage collected each time is intercepted according to the set length and synthesized into a piece of data to obtain the total sample data...
PUM
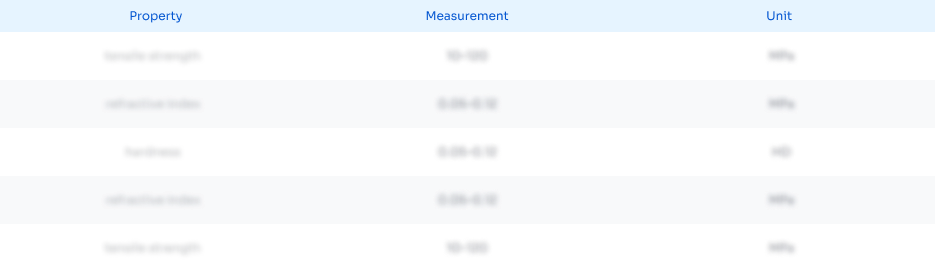
Abstract
Description
Claims
Application Information
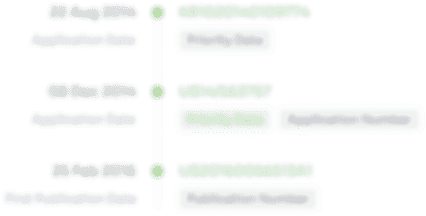
- R&D Engineer
- R&D Manager
- IP Professional
- Industry Leading Data Capabilities
- Powerful AI technology
- Patent DNA Extraction
Browse by: Latest US Patents, China's latest patents, Technical Efficacy Thesaurus, Application Domain, Technology Topic, Popular Technical Reports.
© 2024 PatSnap. All rights reserved.Legal|Privacy policy|Modern Slavery Act Transparency Statement|Sitemap|About US| Contact US: help@patsnap.com