Dynamic non-gaussian process monitoring method based on dynamic latent independent variable
An independent variable, Gaussian process technology, applied in the direction of program control, electrical program control, comprehensive factory control, etc.
- Summary
- Abstract
- Description
- Claims
- Application Information
AI Technical Summary
Problems solved by technology
Method used
Image
Examples
Embodiment Construction
[0050] The method of the present invention will be described in detail below in conjunction with the accompanying drawings and specific examples of implementation.
[0051] The invention discloses a dynamic non-Gaussian process monitoring method based on dynamic latent independent variables, and its off-line modeling implementation process is as follows figure 1 As shown, it specifically includes the following steps:
[0052] (1) Collect samples under the normal operating state of the production process to form a training data matrix X∈R n×m , and calculate the mean μ of each column vector in matrix X 1 , μ 2 ,…, μ m and the standard deviation δ 1 ,δ 2 ,…,δ m , corresponding to the composition mean vector μ=[μ 1 , μ 2 ,…, μ m ] and standard deviation vector δ=[δ 1 ,δ 2 ,…,δ m ].
[0053] (2) According to the formula Standardize the matrix X to get where U ∈ R n×m It is a matrix composed of n identical mean vectors μ, and the elements on the diagonal in the di...
PUM
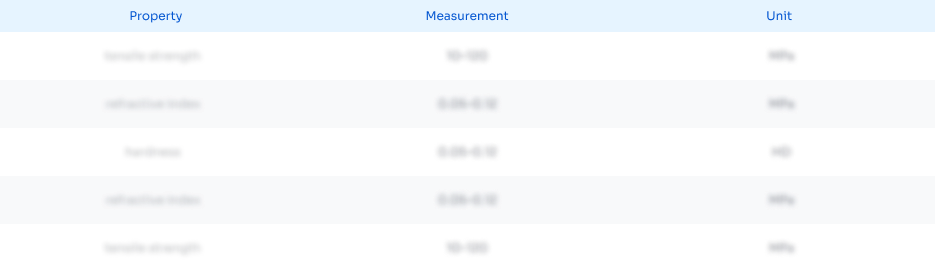
Abstract
Description
Claims
Application Information
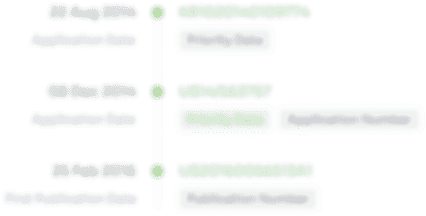
- R&D Engineer
- R&D Manager
- IP Professional
- Industry Leading Data Capabilities
- Powerful AI technology
- Patent DNA Extraction
Browse by: Latest US Patents, China's latest patents, Technical Efficacy Thesaurus, Application Domain, Technology Topic, Popular Technical Reports.
© 2024 PatSnap. All rights reserved.Legal|Privacy policy|Modern Slavery Act Transparency Statement|Sitemap|About US| Contact US: help@patsnap.com