Sgcnn: structural graph convolutional neural network
A convolutional neural network and subgraph technology, applied in the field of SGCNN: structured graph-based convolutional neural network, can solve the problem that the graph kernel is not enough to complete
- Summary
- Abstract
- Description
- Claims
- Application Information
AI Technical Summary
Problems solved by technology
Method used
Image
Examples
Embodiment Construction
[0027] The following disclosure describes the invention in terms of several embodiments related to methods, systems, and apparatus related to structured graph-based convolutional neural networks (SGCNNs) capable of The hierarchy performs graph-invariant learning tasks. The SGCNN architecture performs training tasks at the graph / subgraph level. The architecture provides many other benefits over traditional solutions, including new graph feature compression for graph-based learning based on Node2Vec embeddings; path-based neighbor nodes that aggregate vertex-domain neighbor node information onto subgraphs Ensemble methods; and convolution kernels for subgraphs that can perform graph-invariant convolution operations on graphs or subgraphs.
[0028] In the following disclosure, a graph is defined as G=(V,E), where V is the set of vertices and E is the set of edges. In some embodiments, graph edges may be weighted and directed. However, for simplicity, the following description ...
PUM
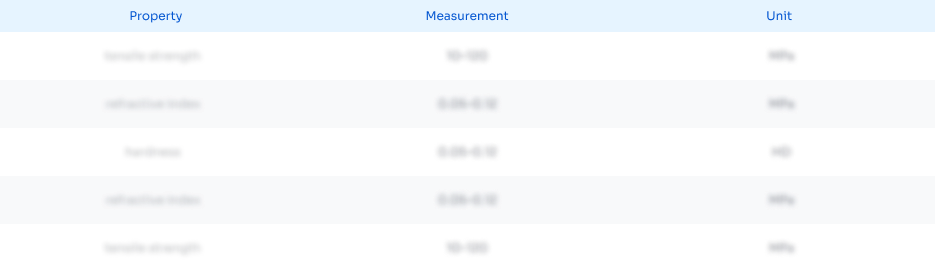
Abstract
Description
Claims
Application Information
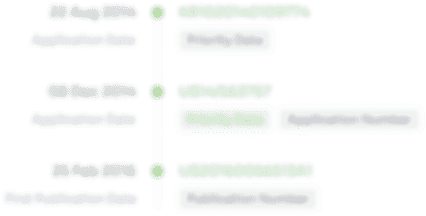
- R&D Engineer
- R&D Manager
- IP Professional
- Industry Leading Data Capabilities
- Powerful AI technology
- Patent DNA Extraction
Browse by: Latest US Patents, China's latest patents, Technical Efficacy Thesaurus, Application Domain, Technology Topic, Popular Technical Reports.
© 2024 PatSnap. All rights reserved.Legal|Privacy policy|Modern Slavery Act Transparency Statement|Sitemap|About US| Contact US: help@patsnap.com