Urban traffic scene image understanding and multi-view crowd-sourcing optimization method
A technology of scene images and urban traffic, applied in the field of urban traffic scene image understanding and multi-view crowd intelligence optimization, can solve problems such as inability to expand and be robust.
- Summary
- Abstract
- Description
- Claims
- Application Information
AI Technical Summary
Problems solved by technology
Method used
Image
Examples
Embodiment Construction
[0033] The present invention is described in further detail below in conjunction with accompanying drawing:
[0034] The urban traffic scene image understanding and multi-view crowd intelligence optimization method specifically includes the following steps:
[0035] Step 1), obtain the three-dimensional information of the vehicle based on the vehicle target, and establish a two-dimensional detection candidate frame of the vehicle target that reflects the prior knowledge of the three-dimensional space; for the structurally incomplete road surface occupied by road traffic participants, the method of deep learning is used to perform semantic analysis on the road surface. Prior modeling to obtain road semantic prior model;
[0036] Step 2), using the image entropy sorting of the candidate frame to realize the region of interest extraction of the vehicle target, and performing the precise regression of the bounding box, the regression of the angle and the score prediction by the regr...
PUM
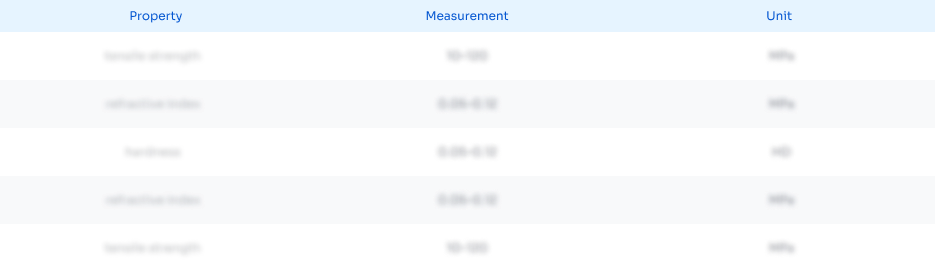
Abstract
Description
Claims
Application Information
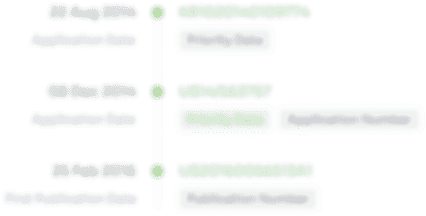
- R&D Engineer
- R&D Manager
- IP Professional
- Industry Leading Data Capabilities
- Powerful AI technology
- Patent DNA Extraction
Browse by: Latest US Patents, China's latest patents, Technical Efficacy Thesaurus, Application Domain, Technology Topic, Popular Technical Reports.
© 2024 PatSnap. All rights reserved.Legal|Privacy policy|Modern Slavery Act Transparency Statement|Sitemap|About US| Contact US: help@patsnap.com