Image enhancement method based on all 1*1 convolution neural network
A convolutional neural network and image enhancement technology, applied in the field of image processing, can solve problems such as high computational cost and limited wide application, achieve good accuracy, light weight CNN structure, and prevent overfitting.
- Summary
- Abstract
- Description
- Claims
- Application Information
AI Technical Summary
Problems solved by technology
Method used
Image
Examples
Embodiment Construction
[0032] The present invention is further analyzed below in conjunction with specific examples.
[0033] The present invention can be used for various image enhancement tasks such as color constancy (color constancy, also called image color shift correction, white balance, etc.), image defogging, low-light image enhancement, and image noise level estimation. In the following, specific implementations of the present invention are mainly introduced for two embodiments of color constancy and image defogging.
[0034] 1 Image preprocessing
[0035] Randomly rearrange the local image blocks or the pixels in the entire image in the low-quality imaging image (PixelShuffle); the random rearrangement of the pixels is local rearrangement or global rearrangement. Pixels in the image, rearranging all their pixel position order randomly. Re-arranging (Pixel Shuffle) image blocks or pixels in the entire image will not change their statistical properties, but will destroy the spatial structu...
PUM
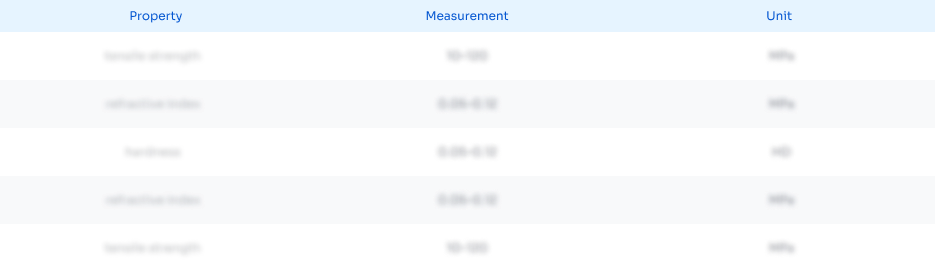
Abstract
Description
Claims
Application Information
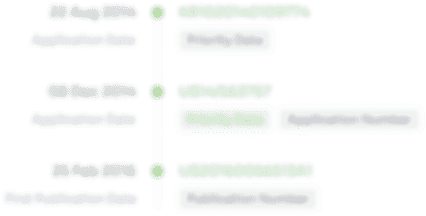
- R&D Engineer
- R&D Manager
- IP Professional
- Industry Leading Data Capabilities
- Powerful AI technology
- Patent DNA Extraction
Browse by: Latest US Patents, China's latest patents, Technical Efficacy Thesaurus, Application Domain, Technology Topic, Popular Technical Reports.
© 2024 PatSnap. All rights reserved.Legal|Privacy policy|Modern Slavery Act Transparency Statement|Sitemap|About US| Contact US: help@patsnap.com