An anomaly detection method for mechanical monitoring data based on kernel estimation LOF
A technology of monitoring data and detection methods, which is applied in computer parts, calculation, pattern recognition in signals, etc.
- Summary
- Abstract
- Description
- Claims
- Application Information
AI Technical Summary
Problems solved by technology
Method used
Image
Examples
Embodiment Construction
[0033] The present invention will be described in further detail below in conjunction with the accompanying drawings.
[0034] Such as figure 1 As shown, a method for abnormal segment detection of mechanical monitoring data based on kernel estimation LOF includes the following steps:
[0035] 1) Use length w 1 The sliding time window of the mechanical monitoring data is divided into N data segments, and the length increment Δw of the sliding time window is defined, so that the overlapping rate of two adjacent windows is 90%, and a set D of data segments is obtained;
[0036] 2) Extract the feature index of each data segment from the time domain, frequency domain, and time-frequency domain respectively to form a feature index set in Indicates that the length of the sliding window is w 1 When is the characteristic index vector of the i-th data segment, the characteristic index includes mean value, maximum value, minimum value, peak-to-peak value, variance, kurtosis, root m...
PUM
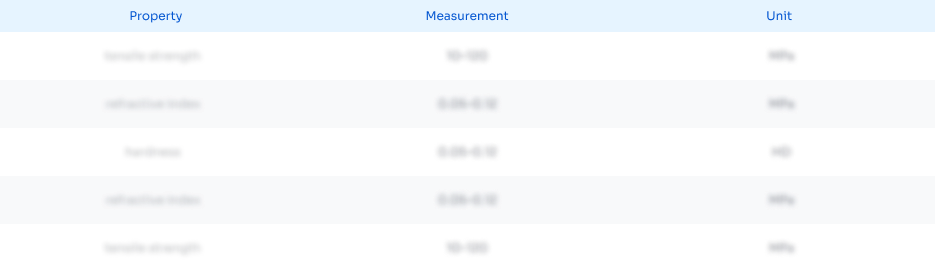
Abstract
Description
Claims
Application Information
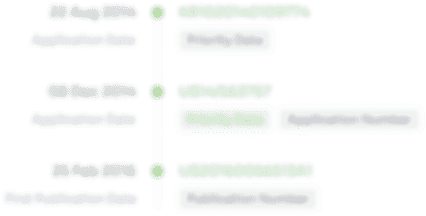
- Generate Ideas
- Intellectual Property
- Life Sciences
- Materials
- Tech Scout
- Unparalleled Data Quality
- Higher Quality Content
- 60% Fewer Hallucinations
Browse by: Latest US Patents, China's latest patents, Technical Efficacy Thesaurus, Application Domain, Technology Topic, Popular Technical Reports.
© 2025 PatSnap. All rights reserved.Legal|Privacy policy|Modern Slavery Act Transparency Statement|Sitemap|About US| Contact US: help@patsnap.com