Joint inversion method of time-frequency electromagnetic and magnetotelluric based on deep learning
A time-frequency electromagnetic and magnetotelluric technology, used in scientific instruments, electrical/magnetic exploration, geophysical measurements, etc.
- Summary
- Abstract
- Description
- Claims
- Application Information
AI Technical Summary
Problems solved by technology
Method used
Image
Examples
Embodiment
[0060] (1) Design technical scheme of input data. The input data consists of three parts:
[0061] a) Magnetotelluric data. For layered media models, use N MT The apparent resistivity and phase data of a measurement point under frequency points are used as inversion data. For the former, take 0.1×lg(ρ a)+0.2 transformation processing, the phase data is divided by 2π for normalization processing.
[0062] b) Frequency domain response data of time-frequency electromagnetic data. for the observed E x Component or H y The component frequency response data is transformed as follows: Among them, n s is the number of measuring points, is the number of frequency points, d o means E x Component or H y Component data, * indicates the conjugate of the complex number, the real part and imaginary part of the processed data d are used as the input data of the two channels of this part.
[0063] c) Time-domain response data of time-frequency electromagnetic data. Take the o...
PUM
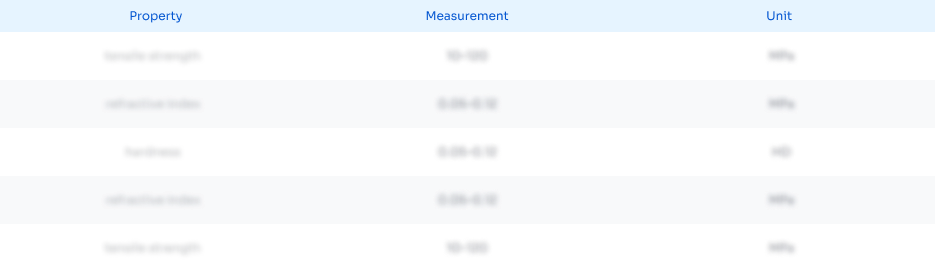
Abstract
Description
Claims
Application Information
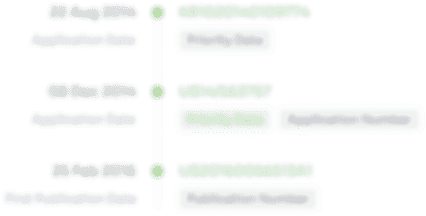
- R&D Engineer
- R&D Manager
- IP Professional
- Industry Leading Data Capabilities
- Powerful AI technology
- Patent DNA Extraction
Browse by: Latest US Patents, China's latest patents, Technical Efficacy Thesaurus, Application Domain, Technology Topic, Popular Technical Reports.
© 2024 PatSnap. All rights reserved.Legal|Privacy policy|Modern Slavery Act Transparency Statement|Sitemap|About US| Contact US: help@patsnap.com