A Robust Fusion Algorithm of ts-rbf Fuzzy Neural Network for Infrared Flame Recognition
A fuzzy neural network and flame recognition technology, applied in the field of infrared flame recognition, can solve the problem that the model cannot suppress the output of outliers, the model uncertainty processing effect is not good, and the outliers cannot be clustered into one class.
- Summary
- Abstract
- Description
- Claims
- Application Information
AI Technical Summary
Problems solved by technology
Method used
Image
Examples
Embodiment Construction
[0113] The technical solutions of the present invention will be further described below according to the drawings and specific embodiments.
[0114] This example is attached figure 2The shown three-band flame detector hardware is based on the experiments done, and the three pyroelectric infrared sensors have different sensitivity factors to different bands of infrared light. The detection bands are selected as 3.8 microns (artificial heat source band), 4.3 microns (flame detection band), and 5.0 microns (background radiation band), and the half-wave bandwidths of the three bands are all 0.2 microns.
[0115] The main hardware structure of the flame detector includes: sensor module, signal amplification and filtering module, A / D sampling module, communication interface module, voltage reference module, microprocessor module, etc., as attached figure 2 and shown in Table 1.
[0116] Table 1 The hardware composition of the detector
[0117]
[0118]
[0119] The data c...
PUM
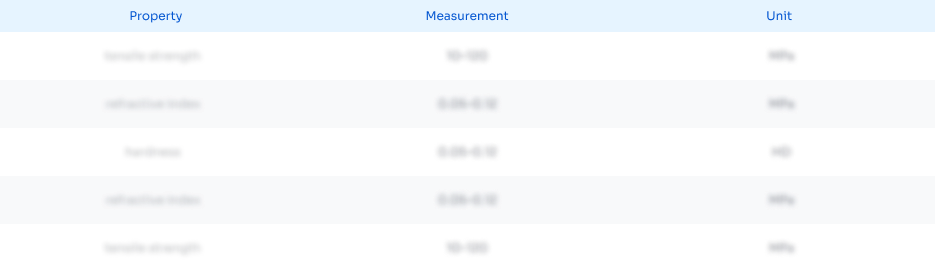
Abstract
Description
Claims
Application Information
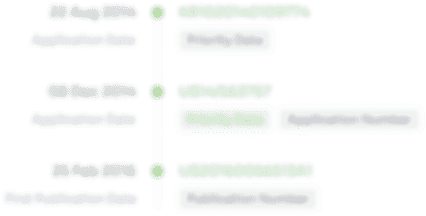
- R&D Engineer
- R&D Manager
- IP Professional
- Industry Leading Data Capabilities
- Powerful AI technology
- Patent DNA Extraction
Browse by: Latest US Patents, China's latest patents, Technical Efficacy Thesaurus, Application Domain, Technology Topic, Popular Technical Reports.
© 2024 PatSnap. All rights reserved.Legal|Privacy policy|Modern Slavery Act Transparency Statement|Sitemap|About US| Contact US: help@patsnap.com