A retinal blood vessel image segmentation method based on a multi-scale feature convolutional neural network
A convolutional neural network and retinal blood vessel technology, applied in biological neural network models, image analysis, image enhancement, etc., can solve problems such as a lot of energy and time, and the impact of subjective experience is large, achieving good results and expanding the receptive field. , the effect of reducing the training parameters
- Summary
- Abstract
- Description
- Claims
- Application Information
AI Technical Summary
Problems solved by technology
Method used
Image
Examples
Embodiment Construction
[0040] The present invention proposes a retinal vessel segmentation method based on a convolutional neural network combined with multi-scale features. First, the retinal image is properly preprocessed, including restrictive contrast adaptive histogram equalization and gamma brightness adjustment. At the same time, we have carried out data amplification for the problem of less retinal image data, and cut and segmented the experimental images, which expands the wide applicability of the present invention. Secondly, by constructing a retinal vessel segmentation network combined with multi-scale features, the present invention introduces the spatial pyramid hole pooling into the encoder-decoder structure convolutional neural network, independently optimizes the model parameters through multiple iterations, and realizes pixel-level retinal vessel automatic segmentation. After the segmentation process, the retinal blood vessel segmentation map is obtained. On the one hand, the enco...
PUM
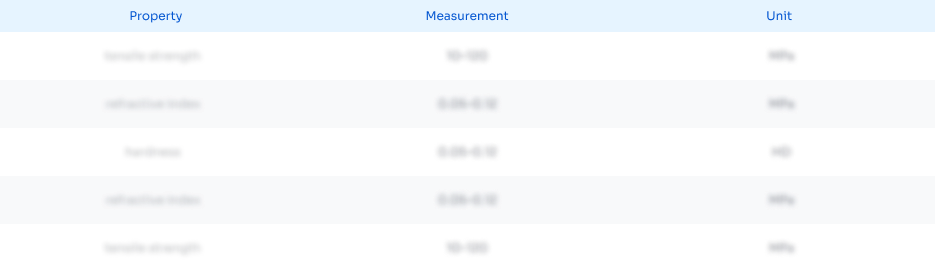
Abstract
Description
Claims
Application Information
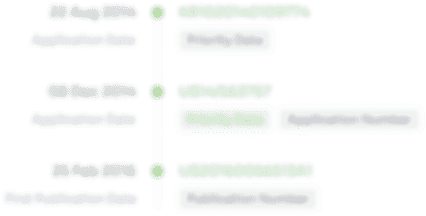
- R&D Engineer
- R&D Manager
- IP Professional
- Industry Leading Data Capabilities
- Powerful AI technology
- Patent DNA Extraction
Browse by: Latest US Patents, China's latest patents, Technical Efficacy Thesaurus, Application Domain, Technology Topic.
© 2024 PatSnap. All rights reserved.Legal|Privacy policy|Modern Slavery Act Transparency Statement|Sitemap