A traffic scene analysis method based on a multi-task network
An analysis method and traffic scene technology, applied in the field of multi-task network design for real-time traffic scene analysis, can solve problems such as poor real-time performance, achieve high accuracy, good real-time performance, and improve segmentation and detection effects
- Summary
- Abstract
- Description
- Claims
- Application Information
AI Technical Summary
Problems solved by technology
Method used
Image
Examples
Embodiment Construction
[0023] Describe the specific embodiment of the present invention in detail below in conjunction with technical scheme and accompanying drawing, a kind of multi-task network design method for real-time traffic scene analysis comprises the following steps:
[0024] A. Multi-task network structure design
[0025] The multi-task network includes encoder, segmentation decoder and detection decoder. The encoder includes a convolutional layer and a downsampling layer, and the convolutional layer adopts a three-layer residual learning unit in a deep residual network, which is used to extract feature information from an original image to obtain a feature map; the described The convolution kernel size of the downsampling layer is 3×3 and the step size is 2, which is used to reduce the size of the feature map; at the end of the encoder, a spatial pyramid pooling layer is included to extract information of different scales in the feature map . Through a hierarchical combination of convo...
PUM
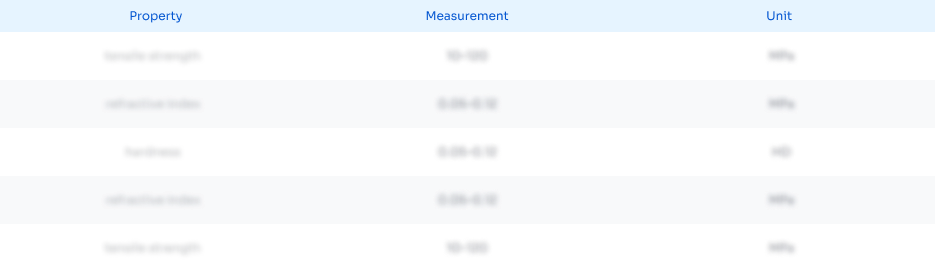
Abstract
Description
Claims
Application Information
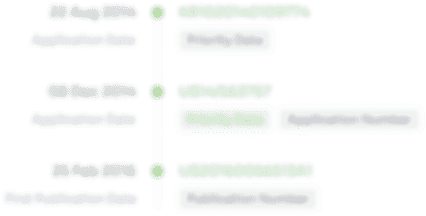
- R&D Engineer
- R&D Manager
- IP Professional
- Industry Leading Data Capabilities
- Powerful AI technology
- Patent DNA Extraction
Browse by: Latest US Patents, China's latest patents, Technical Efficacy Thesaurus, Application Domain, Technology Topic, Popular Technical Reports.
© 2024 PatSnap. All rights reserved.Legal|Privacy policy|Modern Slavery Act Transparency Statement|Sitemap|About US| Contact US: help@patsnap.com