Novel convolutional neural network and Wigner-Ville distribution combined radar signal classification method
A convolutional neural network and radar signal technology, applied in the field of new radar signal classification, can solve problems such as poor stability, unsatisfactory classification accuracy, and large differences in accuracy requirements, and achieve classification accuracy and stability improvement. Effect
- Summary
- Abstract
- Description
- Claims
- Application Information
AI Technical Summary
Problems solved by technology
Method used
Image
Examples
Embodiment Construction
[0025] The embodiments of the present invention are described in detail below. This embodiment is implemented on the premise of the technical solution of the present invention, and detailed implementation methods and specific operating procedures are provided, but the protection scope of the present invention is not limited to the following implementation example.
[0026] Such as Figure 6 As shown, the main steps of this embodiment include: the first step, LPI radar simulation signal generation; the second step, PWVD processing signal data; the third step, constructing a new CNN; the fourth step, using the generated simulation data set to train the new CNN ; The fifth step, the signal classification result output. The specific implementation steps are as follows:
[0027] Step 1, LPI radar simulation signal generation:
[0028] Step 1.1: Generate 10 types (Bpsk, Fmcw, P1, P2, P3, P4, T1, T2, T3, T4) Raw data of LPI radar signal;
[0029] Step 2, PWVD processes signal da...
PUM
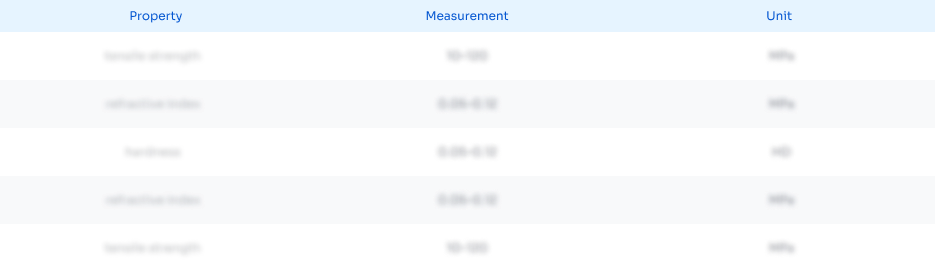
Abstract
Description
Claims
Application Information
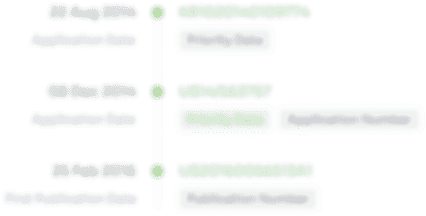
- R&D Engineer
- R&D Manager
- IP Professional
- Industry Leading Data Capabilities
- Powerful AI technology
- Patent DNA Extraction
Browse by: Latest US Patents, China's latest patents, Technical Efficacy Thesaurus, Application Domain, Technology Topic, Popular Technical Reports.
© 2024 PatSnap. All rights reserved.Legal|Privacy policy|Modern Slavery Act Transparency Statement|Sitemap|About US| Contact US: help@patsnap.com