Multi-type entity recognition multi-task deep learning model training method and device
A deep learning and entity recognition technology, applied in the field of multi-task deep learning model training, can solve problems such as high error rate, inability to deeply extract data, and long data extraction process, and achieve overall correctness improvement, accurate identification and extraction. Entity, the effect of good generalization ability
- Summary
- Abstract
- Description
- Claims
- Application Information
AI Technical Summary
Problems solved by technology
Method used
Image
Examples
Embodiment 1
[0043] Such as figure 1As shown, the training method of the multi-task deep learning model of multi-type entity recognition provided by the embodiment of the present invention includes the following steps:
[0044] Step S1, data preprocessing: perform data cleaning operations on all acquired text corpus data according to requirements;
[0045] The preprocessing operation in step S1 is mainly to remove invalid characters, spaces, line breaks, etc. in the text corpus data, or remove the web page format of the text corpus data from the web page, etc., thereby purifying the text data.
[0046] Step S2, establishing a skip-gram neural network model to convert the preprocessed text corpus data into vectors;
[0047] The specific process of step S2 is as follows:
[0048] Use the skip-gram neural network model for training to obtain a fixed character feature vector file named vocb, in which each character is converted into a vector of the same length according to the semantics, the...
Embodiment 2
[0072] Such as image 3 As shown, the present invention provides a multi-task deep learning model training device for multi-type entity recognition, which is used to complete the multi-task deep learning model training method for multi-type entity recognition provided by the present invention. The training setup for the multi-task deep learning model includes:
[0073] Data processing unit for data cleaning: all text corpus data will be obtained and data cleaning will be performed according to the requirements;
[0074] The conversion unit is used to establish the skip-gram neural network model and convert the preprocessed text corpus data into vectors;
[0075] The sample data construction unit is used to establish a data set: the entity type to be identified and extracted is used to construct a sample data set;
[0076] Word segmentation unit: used to construct the word segmentation feature of the sample: the preprocessed text corpus data is segmented according to a single...
PUM
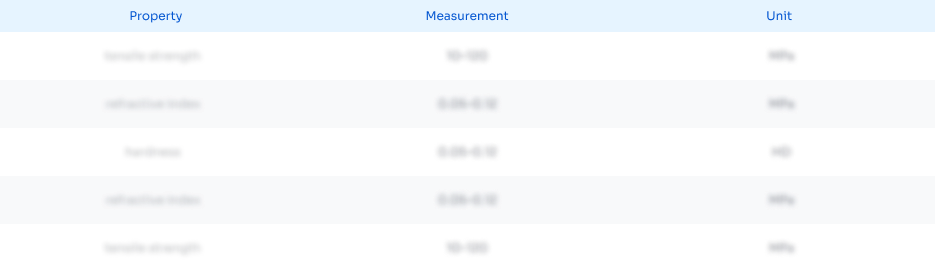
Abstract
Description
Claims
Application Information
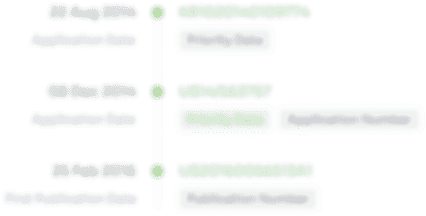
- R&D Engineer
- R&D Manager
- IP Professional
- Industry Leading Data Capabilities
- Powerful AI technology
- Patent DNA Extraction
Browse by: Latest US Patents, China's latest patents, Technical Efficacy Thesaurus, Application Domain, Technology Topic.
© 2024 PatSnap. All rights reserved.Legal|Privacy policy|Modern Slavery Act Transparency Statement|Sitemap