Image classification method based on confrontation network generated through feature recalibration
A classification method and feature map technology, applied in biological neural network models, neural learning methods, instruments, etc., can solve the problems of small training error, poor fitting degree of test set, large test error, etc.
- Summary
- Abstract
- Description
- Claims
- Application Information
AI Technical Summary
Problems solved by technology
Method used
Image
Examples
Embodiment Construction
[0061] The specific embodiment of the present invention will be further described below in conjunction with accompanying drawing:
[0062] Such as figure 1 As shown, the image classification method based on feature recalibration generation confrontation network of the present invention, its steps are:
[0063] S1 constructs a generated confrontational network model, and inputs the image data to be classified into the confrontational network model for network training;
[0064] S2 constructs a generator and a discriminator composed of a convolutional network;
[0065] S3 initializes the random noise, and inputs the random noise into the generator;
[0066] S4 uses the convolutional network in the generator to perform multi-layer deconvolution operations on random noise to finally obtain generated samples;
[0067] S5 will generate samples and input the real samples to the discriminator;
[0068] S6 In the discriminator, the convolutional network is used to perform convoluti...
PUM
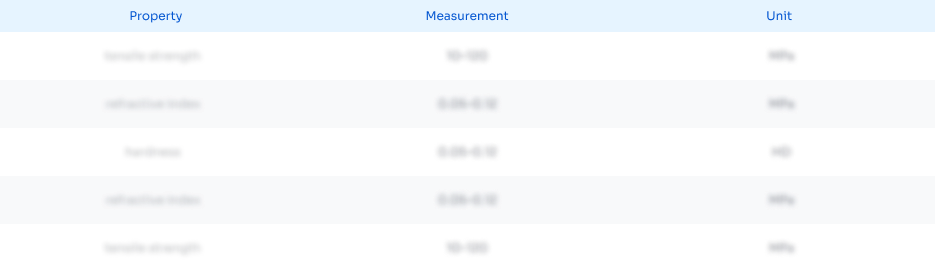
Abstract
Description
Claims
Application Information
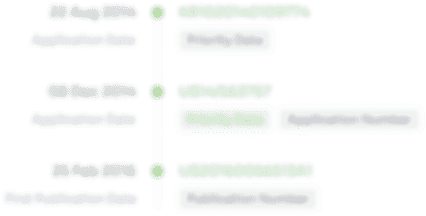
- R&D Engineer
- R&D Manager
- IP Professional
- Industry Leading Data Capabilities
- Powerful AI technology
- Patent DNA Extraction
Browse by: Latest US Patents, China's latest patents, Technical Efficacy Thesaurus, Application Domain, Technology Topic, Popular Technical Reports.
© 2024 PatSnap. All rights reserved.Legal|Privacy policy|Modern Slavery Act Transparency Statement|Sitemap|About US| Contact US: help@patsnap.com