Objective evaluation method for full reference image quality based on neural network learning integration
A neural network learning and objective evaluation method technology, applied in the field of image processing, can solve the problems of lack of experimental results, single information processing algorithm, and difficulty in revealing the working mechanism of the visual brain, achieving good stability and improving performance
- Summary
- Abstract
- Description
- Claims
- Application Information
AI Technical Summary
Problems solved by technology
Method used
Image
Examples
Embodiment Construction
[0053] In order to make the object, technical solution and advantages of the present invention clearer, the present invention will be further described in detail below in conjunction with the accompanying drawings and embodiments. It should be understood that the specific embodiments described here are only used to explain the present invention, and do not limit the protection scope of the present invention.
[0054] In this implementation, the LIVE Release 2 image standard database provided by the image video engineering of the University of Texas at Austin is taken as an example for illustration. The LIVERelease 2 image standard database provided by the Image Video Engineering of the University of Texas at Austin stores some paired standard cases (ie, reference image and distorted image pair), and the distorted image in each case has a corresponding MOS value ( Subjective evaluation score) is known, and the MOS value is the subjective test result of the human eye.
[0055] ...
PUM
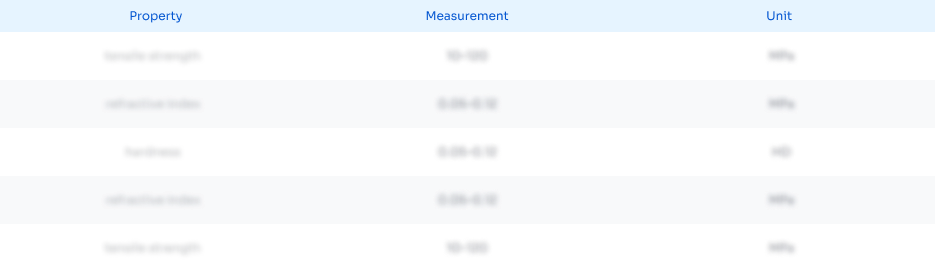
Abstract
Description
Claims
Application Information
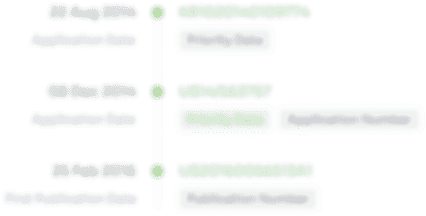
- Generate Ideas
- Intellectual Property
- Life Sciences
- Materials
- Tech Scout
- Unparalleled Data Quality
- Higher Quality Content
- 60% Fewer Hallucinations
Browse by: Latest US Patents, China's latest patents, Technical Efficacy Thesaurus, Application Domain, Technology Topic, Popular Technical Reports.
© 2025 PatSnap. All rights reserved.Legal|Privacy policy|Modern Slavery Act Transparency Statement|Sitemap|About US| Contact US: help@patsnap.com