Optical Remote Sensing Image Retrieval Method Based on Deep Convolutional Semantic Web
A technology of optical remote sensing image and depth convolution, applied in the field of image processing, can solve the problems of unreasonable solution to quantization error, weak semantic information extraction function, weak image retrieval ability, etc., to improve retrieval accuracy and overcome retrieval ability The effect of weak and enhanced image feature expression ability
- Summary
- Abstract
- Description
- Claims
- Application Information
AI Technical Summary
Problems solved by technology
Method used
Image
Examples
Embodiment Construction
[0049] The present invention will be described in further detail below in conjunction with the accompanying drawings.
[0050] Refer to attached figure 1 , the steps of the present invention are further described in detail.
[0051] This method uses the trained deep convolutional semantic network to extract shallow feature vectors, transition feature vectors, and deep feature vectors from optical remote sensing images, and weights and fuses the three extracted feature vectors as the fusion of each image. feature vector, and establish a feature library; respectively calculate the Euclidean distance between the fusion feature vector of the optical remote sensing image to be retrieved and the fusion feature vector of each image in the feature library, as the similarity.
[0052] Step 1, construct a deep convolutional semantic network.
[0053] Build a 25-layer deep convolutional semantic network, the structure of which is: input layer → first convolutional layer → second convol...
PUM
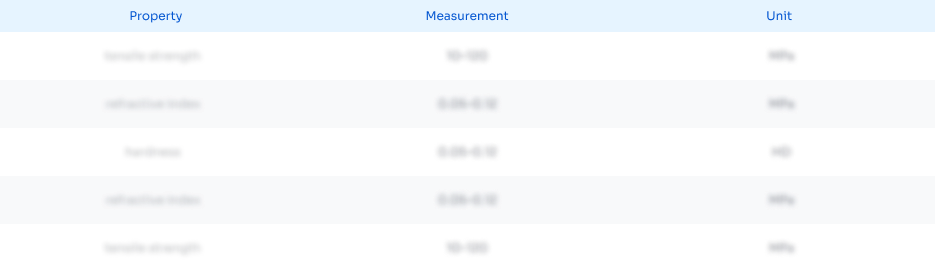
Abstract
Description
Claims
Application Information
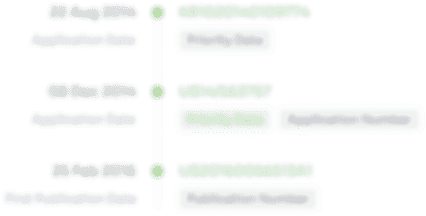
- Generate Ideas
- Intellectual Property
- Life Sciences
- Materials
- Tech Scout
- Unparalleled Data Quality
- Higher Quality Content
- 60% Fewer Hallucinations
Browse by: Latest US Patents, China's latest patents, Technical Efficacy Thesaurus, Application Domain, Technology Topic, Popular Technical Reports.
© 2025 PatSnap. All rights reserved.Legal|Privacy policy|Modern Slavery Act Transparency Statement|Sitemap|About US| Contact US: help@patsnap.com