Method for optimizing multi-kernel multi-feature fusion support vector machine and identifying bearing fault
A support vector machine and multi-feature fusion technology, applied in the field of self-adjusting particle swarm optimization multi-core multi-feature fusion support vector machine, can solve the problems of cumbersome sensor installation and lack of multiple measurement positions, and achieve high fault identification accuracy , Reasonable design, high precision effect
- Summary
- Abstract
- Description
- Claims
- Application Information
AI Technical Summary
Problems solved by technology
Method used
Image
Examples
Embodiment 1
[0039] see figure 1 , figure 2 , image 3 , the present invention optimizes the multi-core multi-feature fusion support vector machine method for bearing fault identification, the steps are as follows: S1. Select the bearing vibration signal collected under a single sensor;
[0040] S2. Decompose the bearing vibration signals at different speeds through EMD (full name Empirical Mode Decomposition, empirical mode decomposition algorithm) to obtain IMF (full name Intrinsic Mode Function, eigenmode component) energy entropy and IMF permutation entropy;
[0041] S3. Fusion of IMF energy entropy and IMF permutation entropy under different rotational speeds is extracted to obtain fusion features containing different rotational speed information, which are used as support vector machine training samples to obtain multi-core multi-feature fusion support vector machines suitable for fault identification under different rotational speeds ;
[0042] S4. Integrating the performance of...
Embodiment 2
[0046] The optimized multi-core multi-feature fusion support vector machine of the present embodiment is used for the method of bearing fault identification, and the difference with embodiment 1 is: in step S4, multi-core least squares support vector machine construction process and parameter optimization process are as follows:
[0047] Select the appropriate Gaussian radial basis function kernel parameter g, polynomial kernel parameter c and combined kernel coefficient p, Gaussian radial basis function kernel: K g =exp(-g·||x-x i || 2 ); the polynomial kernel is defined as: K d =(x T x i +1) c ; Combined kernel function: K=p·(x T x i +1) c +(1-p)·exp(-g·||x-x i || 2 ); through SRPSO optimization parameters p, c, g, support vector machine identification accuracy as the fitness index, the greater the fitness index, the higher the fault identification accuracy; set parameters p, c, g as SRPSO particles, the optimization steps are as follows:
[0048] 1) Initialize the...
Embodiment 3
[0054] In this embodiment, the multi-core multi-feature fusion support vector machine is optimized, and the process for bearing fault identification is as follows: figure 1 shown. The essence of self-adjusting particle swarm optimization multi-core multi-feature fusion support vector machine used in support vector machine is the linear regression that maps samples from nonlinear function space to high-dimensional space, so that samples can be classified according to different characteristics, such as figure 2 shown. Support vector machines are used in pattern recognition processes such as image 3 It can be seen that the important indicators of the difference in recognition accuracy of support vector machines are feature selection and parameter selection.
[0055] For feature selection, S1. Select the bearing vibration signal collected under a single sensor; S2. Decompose the bearing vibration signal at different speeds through EMD to obtain IMF energy entropy and IMF arran...
PUM
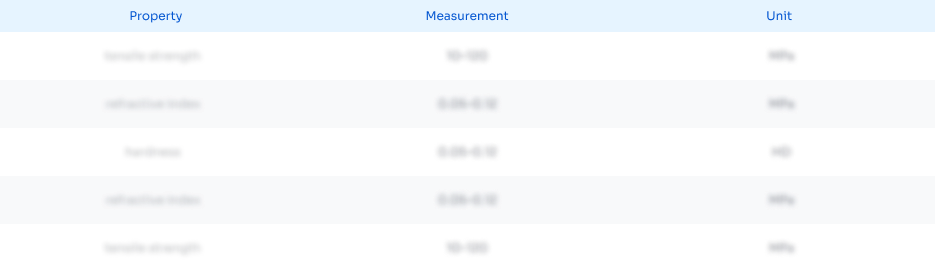
Abstract
Description
Claims
Application Information
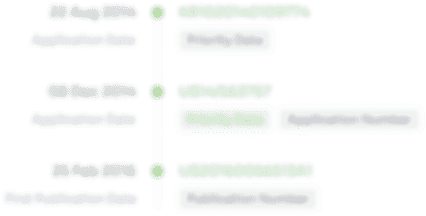
- R&D
- Intellectual Property
- Life Sciences
- Materials
- Tech Scout
- Unparalleled Data Quality
- Higher Quality Content
- 60% Fewer Hallucinations
Browse by: Latest US Patents, China's latest patents, Technical Efficacy Thesaurus, Application Domain, Technology Topic, Popular Technical Reports.
© 2025 PatSnap. All rights reserved.Legal|Privacy policy|Modern Slavery Act Transparency Statement|Sitemap|About US| Contact US: help@patsnap.com