Electrocardio-beat feature automatic extraction and classification methods based on deep learning method
A technology of deep learning and classification methods, applied in medical science, sensors, diagnostic recording/measurement, etc., can solve problems such as inability to extract and classify ECG signals, limited learning ability of traditional models, and rely on doctors for disease diagnosis to achieve simplified features Effects of extraction procedure, precise classification of ECG signals, and simplification of the procedure part
- Summary
- Abstract
- Description
- Claims
- Application Information
AI Technical Summary
Problems solved by technology
Method used
Image
Examples
Embodiment 1
[0039] An automatic extraction method of ECG beat features based on deep learning method, including the following steps: 1) Using biorthogonal wavelet transform to remove high-frequency noise and baseline drift; 2) Using binary spline wavelet transform to generate maxima and minima 3) According to step 2) to detect the QRS complex and P, T waves on the basis of the R wave.
[0040] In this embodiment, the specific detection method of the R wave in the step 3) is: identify the type of the i-th heartbeat, call the i-th heartbeat the current heartbeat and denote it as C-B; denote the i-1th heartbeat as P-B; record the i+1th beat as N-B; the peak positions of R waves of C-B, P-B, and N-B are respectively R i-1 , R i , R i+1 ; i-1 , R i The time difference, that is, the RR interval of the current heartbeat, is recorded as C-RR; R i-1 , R i-1 The time difference, that is, the RR interval of the i-1th heartbeat, is recorded as P-RR; R i+1 , R i The time difference, that is, t...
Embodiment 2
[0050] An automatic classification method of ECG beat features based on the deep learning method, through the binary spline wavelet transform to generate the maximum and minimum values of the detected waveform, using the bidirectional long-term short-term memory network (Bi-LSTM) to deeply analyze the detected waveform data information Learn to classify.
[0051] In this embodiment, each training sequence in the two-way long-short-term memory network (Bi-LSTM) is composed of two long-short-term memory networks (LSTM) connected together by the same output layer, respectively forward long-short-term memory network and backward long-term short-term memory network; the two-way long-term short-term memory network (Bi-LSTM) provides complete past and future context information for each point in the input sequence of the output layer. In this process, each time step There are six unique weights that are reused, namely, the weight w1 from the input layer to the forward hidden layer,...
PUM
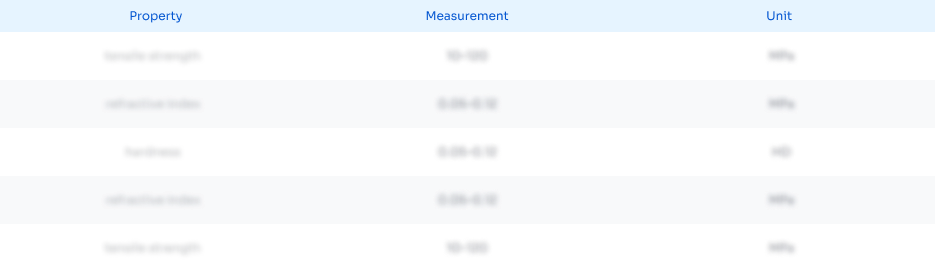
Abstract
Description
Claims
Application Information
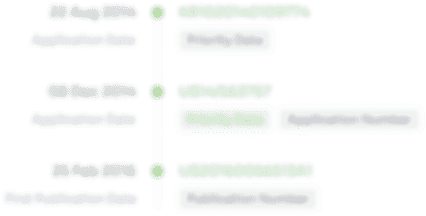
- R&D Engineer
- R&D Manager
- IP Professional
- Industry Leading Data Capabilities
- Powerful AI technology
- Patent DNA Extraction
Browse by: Latest US Patents, China's latest patents, Technical Efficacy Thesaurus, Application Domain, Technology Topic, Popular Technical Reports.
© 2024 PatSnap. All rights reserved.Legal|Privacy policy|Modern Slavery Act Transparency Statement|Sitemap|About US| Contact US: help@patsnap.com