Target detection algorithm based on multi-feature extraction and multitask fusion
A target detection algorithm and target detection technology, applied in the field of target detection algorithms based on multi-feature extraction and multi-task fusion, can solve the problems of inaccurate positioning, fixed network input size, slow training speed, etc., to achieve reasonable design and improve detection Accuracy and stability, the effect of accurate classification
- Summary
- Abstract
- Description
- Claims
- Application Information
AI Technical Summary
Problems solved by technology
Method used
Image
Examples
Embodiment Construction
[0031] Embodiments of the present invention will be described in further detail below in conjunction with the accompanying drawings.
[0032] A target detection algorithm based on multi-feature extraction and multi-task fusion is in figure 1 Implemented on the given target detection framework, it improves target detection performance through multi-feature extraction and multi-task fusion methods. Its design idea is as follows: firstly, image features are extracted based on the deep learning VGG-16 convolutional neural network architecture, and the output results of the 1st, 3rd and 5th layers of convolution are extracted to form a multi-feature map, and the area of the target region of interest is extracted on the feature map. 1, 2, and 3 times the feature area to obtain different visual fields of interest, and then perform feature connection on the extraction results; secondly, implement semantic segmentation on the original image to extract the target segmentation area res...
PUM
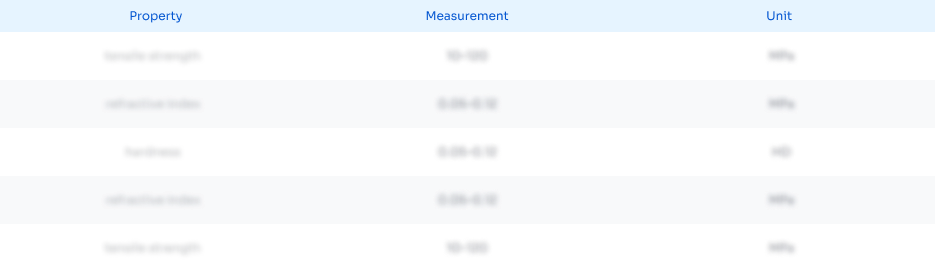
Abstract
Description
Claims
Application Information
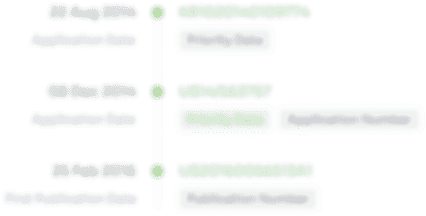
- R&D Engineer
- R&D Manager
- IP Professional
- Industry Leading Data Capabilities
- Powerful AI technology
- Patent DNA Extraction
Browse by: Latest US Patents, China's latest patents, Technical Efficacy Thesaurus, Application Domain, Technology Topic, Popular Technical Reports.
© 2024 PatSnap. All rights reserved.Legal|Privacy policy|Modern Slavery Act Transparency Statement|Sitemap|About US| Contact US: help@patsnap.com