GBM multimodal magnetic resonance image segmentation method based on deep neural network
A technology of deep neural network and magnetic resonance image, which is applied in biological neural network model, neural learning method, image analysis, etc., can solve the problems of inability to speed up the segmentation speed and the explosion of network weights, and avoid the explosion of network weights , Speed up the segmentation speed, and strengthen the effect of independent learning ability
- Summary
- Abstract
- Description
- Claims
- Application Information
AI Technical Summary
Problems solved by technology
Method used
Image
Examples
Embodiment 1
[0072] Embodiment 1, GBM multimodal magnetic resonance image segmentation method based on deep neural network, such as Figure 1-Figure 5 shown, including the following:
[0073] The GBM multimodal magnetic resonance image is segmented to obtain slice images, and each slice image is preprocessed to extract the image block, and then the trained deep neural network segmentation model is used to divide the 8× centered image block center voxel. All the voxels in the 8 area are classified, and the category of the voxel point is determined to be the normal tissue area of the brain (hereinafter referred to as C 0 ), necrotic area (hereinafter referred to as C 1 ), edema area (hereinafter referred to as C 2 ), non-enhancing tumor area (hereinafter referred to as C 3 ) or enhanced tumor area (hereinafter referred to as C 4 ), so as to complete the segmentation of the four modality volume images of GBM, and the result of the post-processing is the final segmentation result of the ...
PUM
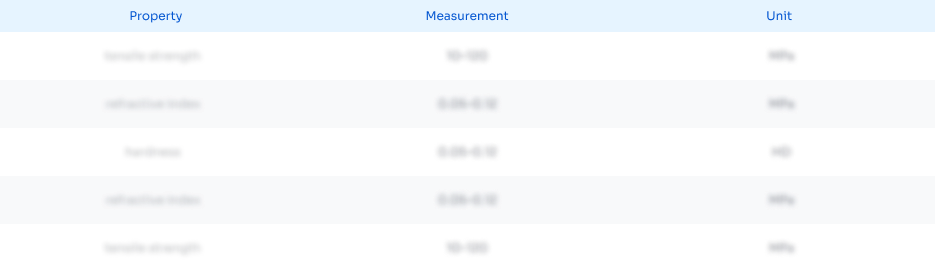
Abstract
Description
Claims
Application Information
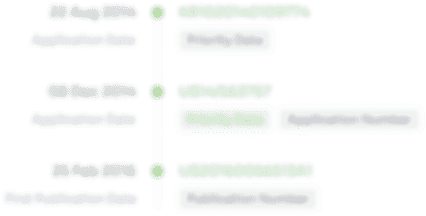
- R&D Engineer
- R&D Manager
- IP Professional
- Industry Leading Data Capabilities
- Powerful AI technology
- Patent DNA Extraction
Browse by: Latest US Patents, China's latest patents, Technical Efficacy Thesaurus, Application Domain, Technology Topic, Popular Technical Reports.
© 2024 PatSnap. All rights reserved.Legal|Privacy policy|Modern Slavery Act Transparency Statement|Sitemap|About US| Contact US: help@patsnap.com